One of the most exciting potential uses of artificial intelligence (AI) is how generative AI search will influence traditional search. The Google search bar we’ve become familiar with (and replicated across various websites) has restrictions generative AI search does not. Generative AI search can synthesize data and deliver proactive feedback — revolutionizing the way we search for information both on the internet and within programs.
In this blog, we will explore the impact of generative AI on search, highlight its benefits, and discuss real-world applications across various industries. And in case you’re wondering — it’s not likely Google will be dethroned, but its search functionality will likely adapt to this new technology.
[/et_pb_text][et_pb_text admin_label="Generative AI Search vs. Traditional Search" _builder_version="4.23.1" _module_preset="default" global_colors_info="{}" theme_builder_area="post_content"]Generative AI Search vs. Traditional Search
Traditional search engines rely on keyword-based queries. Anyone who’s run into the initialism “SEO” (search engine optimization) knows the many ways websites are designed around emphasizing a keyword to communicate to Google (the search engine) their page is relevant for specific searches. This has “worked” better than any other alternative, but it has its weaknesses.
Keyword-based search naturally leads to a flood of irrelevant information. Advanced search engines (like Google) have found a way to resolve some common problems, but if you’ve ever had to rely on a search functionality within a website you’ve probably been exposed to these challenges in keyword search. Imagine you visit the country of Poland and discovered a specific brand that you want to purchase. You don’t remember the name so you search “Polish shoes” or maybe “Polish shoes brands.” These keywords are indistinguishable from “Shoe polish brands,” which obviously bring up entirely different results. Companies like Google have found a way to resolve this specific problem, but if you’re utilizing search within your own database you will likely experience this problem.
Generative AI search doesn’t have this problem. Gen AI understands natural language, context, and these abilities enable it to deliver more accurate and relevant search results. If you’re searching for one specific product, Gen AI can analyze the context of your conversation and narrow results based on that analysis. To use our previous example, you wouldn’t need to overthink how to string together the keywords “Polish shoe brands,” you could simply ask “I’m looking for a Polish shoe brand. I just visited the country a few weeks ago but don’t remember the name.” The Gen AI can understand the context of this prompt and guide you in the right direction. This proactive and personalized search experience results in a better user experience and saves time by quickly providing the most relevant information.
[/et_pb_text][et_pb_accordion _builder_version="4.23.1" _module_preset="default" global_colors_info="{}" theme_builder_area="post_content"][et_pb_accordion_item open="on" _builder_version="4.23.1" _module_preset="default" global_colors_info="{}" theme_builder_area="post_content"]What’s the difference between GenAI and an LLM?
Generative artificial intelligence broadly refers to artificial intelligence that is capable of generating content. Tools like Chat-GPT are just as much “Gen AI” as another tool like Midjourney — even though the latter only produces images, no text feedback whatsoever. A large language model (LLM) has a narrower focus on just language or text-based responses. In other words, an LLM is a type of GenAI, but GenAI is more than just an LLM. We have an entire blog answering the question “what is an LLM?”
[/et_pb_accordion_item][/et_pb_accordion][et_pb_text admin_label="1 line" _builder_version="4.16" _module_preset="default" global_colors_info="{}" theme_builder_area="post_content"]This distinction between traditional search and generative ai search suggests some promising use cases across different industries. We’ve selected a few examples below.
[/et_pb_text][et_pb_text admin_label="body" _builder_version="4.23.1" _module_preset="default" global_colors_info="{}" theme_builder_area="post_content"]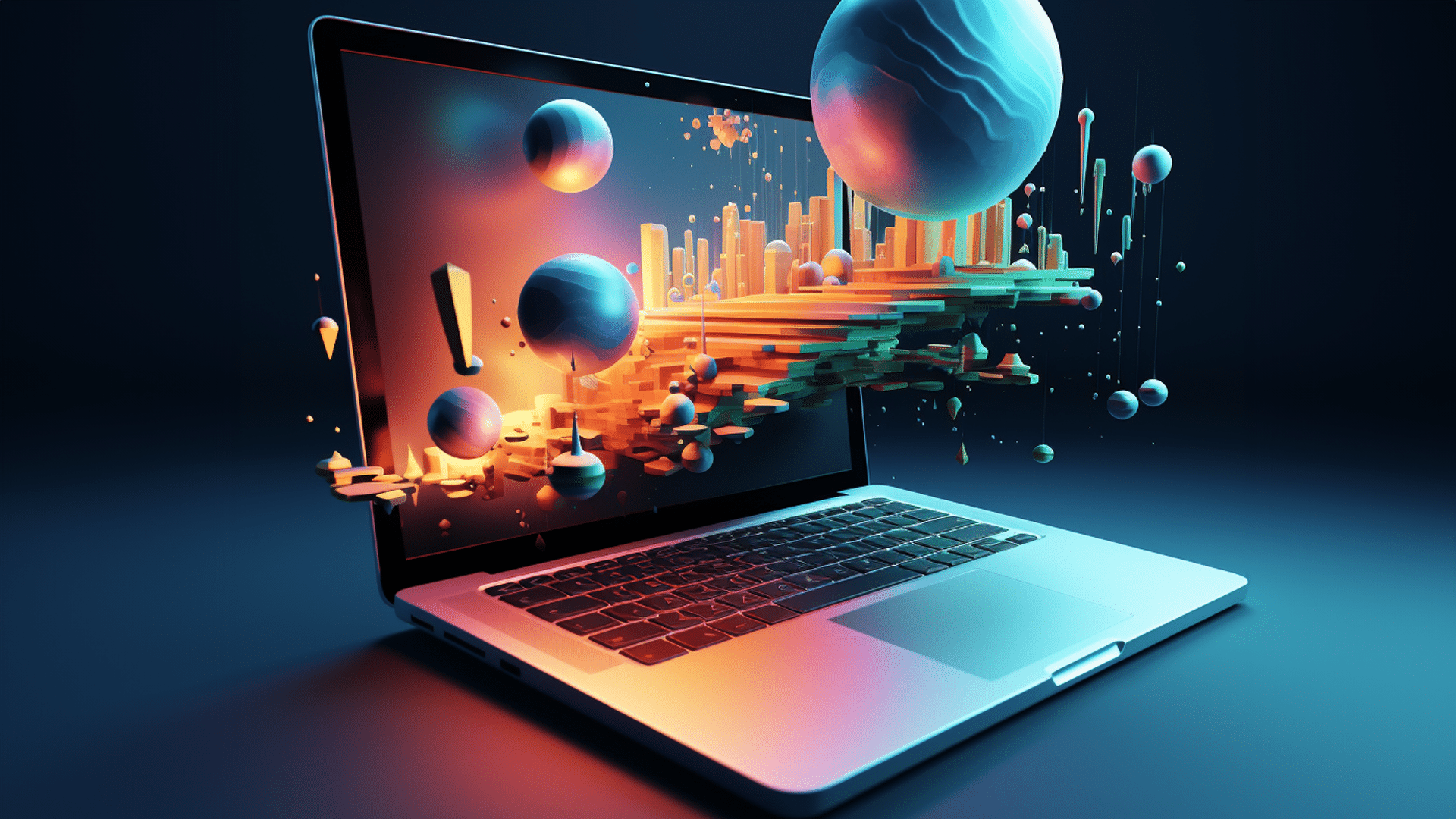
Gen AI Has Revolutionized Customer Service:
One of the early winners of generative AI search has been customer service and contact centers. Contact centers are measured by metrics such as average handle time, time spent looking for answers, and accuracy of information provided. Customer service representatives perform best when they have easy access to knowledge that can resolve customer queries. Sometimes an organization’s knowledge is difficult to access due to technology, or maybe the amount of knowledge is so large it’s challenging to navigate (such as an insurance company and its various policies).
Generative AI search can analyze a customer’s query, retrieve relevant information based on their question, and instantly generate a synthesized response. This response is provided to the customer service representative who can review the information and ensure its relevancy before providing it to the customer. Some AI search tools will even cite the document that informed the generated response. A representative can use this citation to verify information or explore why a generated response might be incorrect.
The functionality of generative AI search has revolutionized contact centers. Customers who have experienced the speed and accuracy of service augmented by AI now have the expectation other service experiences should meet that standard. It’s a high standard to meet, but it’s just as beneficial to contact centers. We’ve seen contact centers reduce average handle time, increase customer satisfaction, and save on onboarding costs thanks to ai generative search.
Gen AI Search Can Advance Medical Research:
Another field ideal for generative ai search is medical research. Any traditional search intended to find specific research has always been a nightmare. Research papers are not written to be easily understood by search engines. The sheer amount of text within any paper — in addition to the obscenely long titles and half-dozen co-authors — make it immune to traditional search engines’ attempts to make them more findable through keywords.
Researchers can leverage generative ai search to analyze vast amounts of medical literature and extract relevant information. Assuming the Gen AI doesn’t suffer from any accuracy challenges, it can even summarize long research and enable users to decide what sections they want to expand. Enabling medical professionals to digest research meaningfully accelerates the process of discovering new treatments, identifying patterns in patient data, and predicting disease outcomes.
For example, generative AI can assist researchers identify potential drug interactions, analyze genomic data, or suggest personalized treatment plans based off of prior data. These benefits apply to any science field that heavily relies on vast amounts of research to inform future decision-making. For the layperson outside of the medical field, tracking the prevalence of medical breakthroughs is one way of tracking the extent of AI’s influence on society.
Other Real-World Applications
The two above examples can (or have) radically alter their respective industries, but there are other applications of Gen AI that will provide a more modest benefit. For example, e-commerce companies are utilizing generative AI to enhance production recommendations. Amazon specifically rolled out generative AI to summarize reviews to expedite customer buying decisions. Generative AI search can also assist customers search for the specific product they need.
The potential for generative AI can influence other sectors with knowledge workers, but it may take time for the industry to accept the technology. Financial institutions are already leveraging generative AI to analyze market trends and provide investment advice. Law firms have explored using generative AI search to assist lawyers retrieve relevant case law and summarize arguments from prior cases. Both of these applications could be revolutionary, so long as they convince the MBA and JD graduates of the accuracy of its outputs.
Generative AI Search is Changing Things
Generative AI is revolutionizing search by delivering personalized and context-aware search experiences. This technology has revolutionized customer service with greater efficiency and accuracy. It has the potential to advance medical research by providing more relevant results for searches of prior studies and papers. Gen AI is also positioned to be widely used to expedite financial recommendations or summarize legal arguments within the next few years. As this technology continues to evolve, we can expect even more innovative applications and improvements in search capabilities, opening up new possibilities across industries.
[/et_pb_text][et_pb_text admin_label="Outro" _builder_version="4.16" _module_preset="default" global_colors_info="{}" theme_builder_area="post_content"]