Semantic search goes far beyond the words that people use in their searches, to interpret the intent behind the words, and the greater context in which people are asking. Traditional lexical or keyword-based technologies cannot accomplish this. The relevance and actionability of information that semantic search retrieves are far superior, as is its ability to serve a wide variety of organizational goals that ladder up to revenue, customer, and employee satisfaction.
Understanding how semantic search technologies integrate into our IT, enterprise data, and content ecosystem is crucial. It will accelerate the adoption and value realization of our technology investments and facilitate powerful AI integrations.
This article will address the following questions:
- What is semantic search?
- How is semantic search different from lexical or keyword search?
- What are the implications of semantic search for various business types and use cases?
Semantic Search and the Future of Information Creativity in the AI Era
We have all witnessed a paradigm shift in how we search, interpret, and create with information. Semantic search technologies are integral to this transformation. While lexical or keyword-based search relies on matching the words you type to the words that are present in documents and metadata, semantic search goes deeper than just keywords and their synonyms to predict the user’s intent and better understand the information to retrieve. It delves deeper into the context and meaning behind user queries, and offers a more intuitive and relevant search experience. For example, consider a doctor searching for 'Pharmacokinetics of long-acting antipsychotic medications for anxiety in nursing home women’.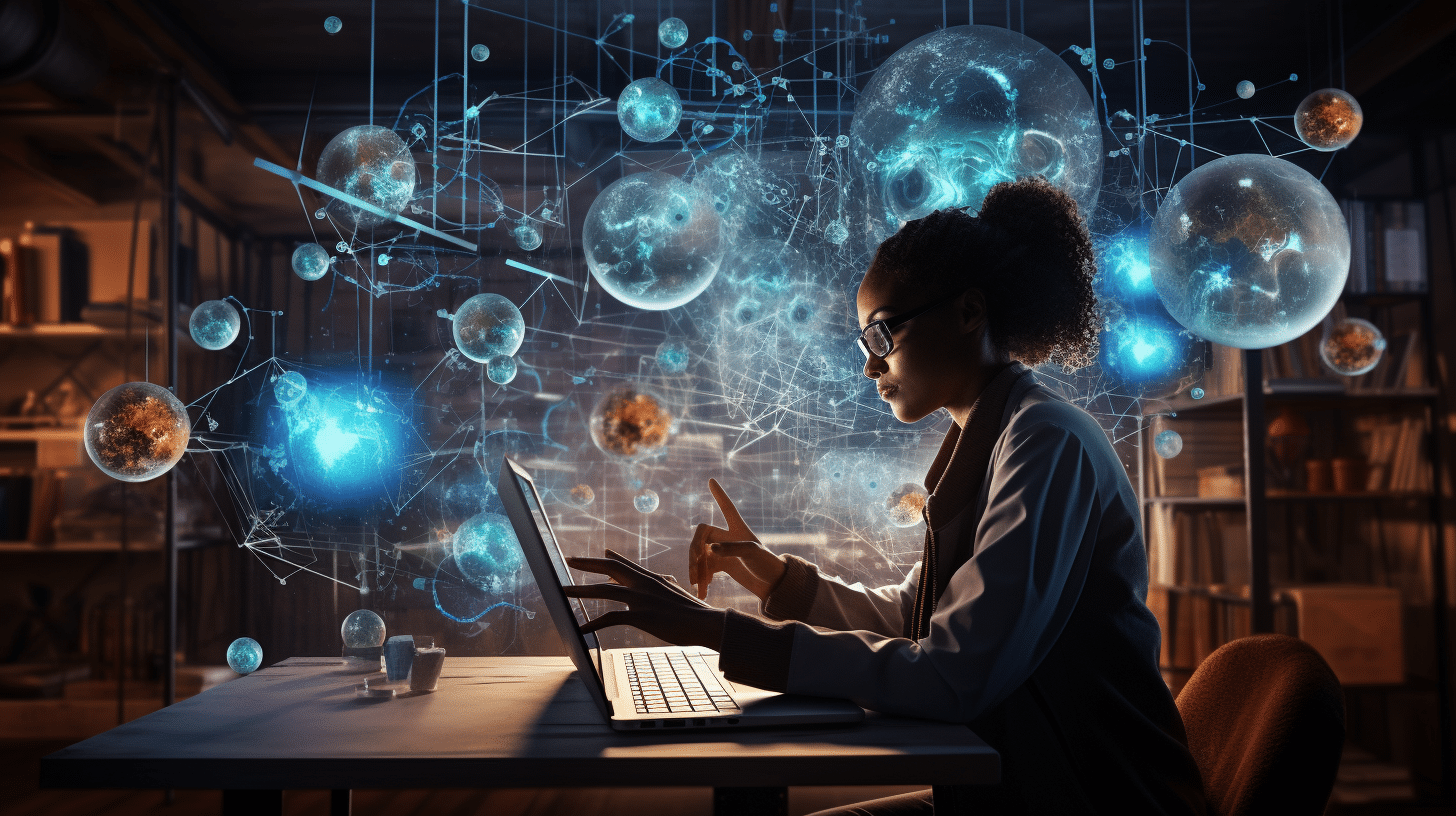
What is Semantic Search?
The term "semantic" has its roots in the Greek word "semantikos," which means "significant" or "having meaning." "Semantic" generally refers to the meaning or interpretation of words, phrases, visual symbolism, or actions that contain symbolic meaning. When we talk about something being 'semantic', we're focused on the contextual meaning behind the words, not just the words themselves. For example, if someone says, “I’m feeling blue today,” we understand they mean they're feeling sad, not that they've turned the color blue. If someone feels blue, you’d hand them a tissue and express empathy. If someone is blue, you’d call an ambulance. The difference is a semantic difference. Consequently, at its core, semantic search transcends the limits of face-value keywords in order to better understand the broader context of the searcher’s intent. This has decisive implications for the true meaning of words and for what information is actually relevant to present. The search employs Natural Language Processing (NLP), machine learning, and sophisticated algorithms to interpret and predict user intent via contextual meaning of the search terms and signals from other data sources, all matched to the contextual meaning of information that is retrievable and that, additionally, systems can execute programmatic operations upon if desired. Let’s dive deeper into the difference between semantic and lexical/keyword search, and see how this difference plays out in various business contexts.How is Semantic Search Different from Lexical or Keyword Search?
Basic Keyword Search: A Rudimentary Matchmaking Game
Basic lexical keyword search involves matching the exact words or phrases (keywords) that a user types into the search bar with those found in the database. Keyword search primarily relies on the frequency and location of specific keywords within their indexed content, often returning results that match these terms without considering the context or meaning. This approach can lead to results that are technically and literally correct but may not always align with the user's actual intent. In order for the search experience to become more impactful, the system must have a better understanding of the context of the search and of the retrievable content. That is, the semantic context.The Semantic Search Difference: Context and Intent
A semantic approach represents a more advanced, contextually aware approach. It goes beyond the literal matching of keywords to understand the intent and contextual meaning behind a user's query. Semantic search embodies an understanding that words are more than just a collection of letters; they are symbols of ideas and concepts that only reveal their true meaning when considered within the broader context of language and human interaction. Utilizing technologies like Natural Language Processing (NLP) and machine learning, it interprets the nuances of human language, including synonyms, variations, and the relationship between words. Systems may also contextually use profiling data such as the user's past search behavior, content interactions, network and organization affiliations, or other profiling data. This leads to more accurate, relevant, and personalized search results.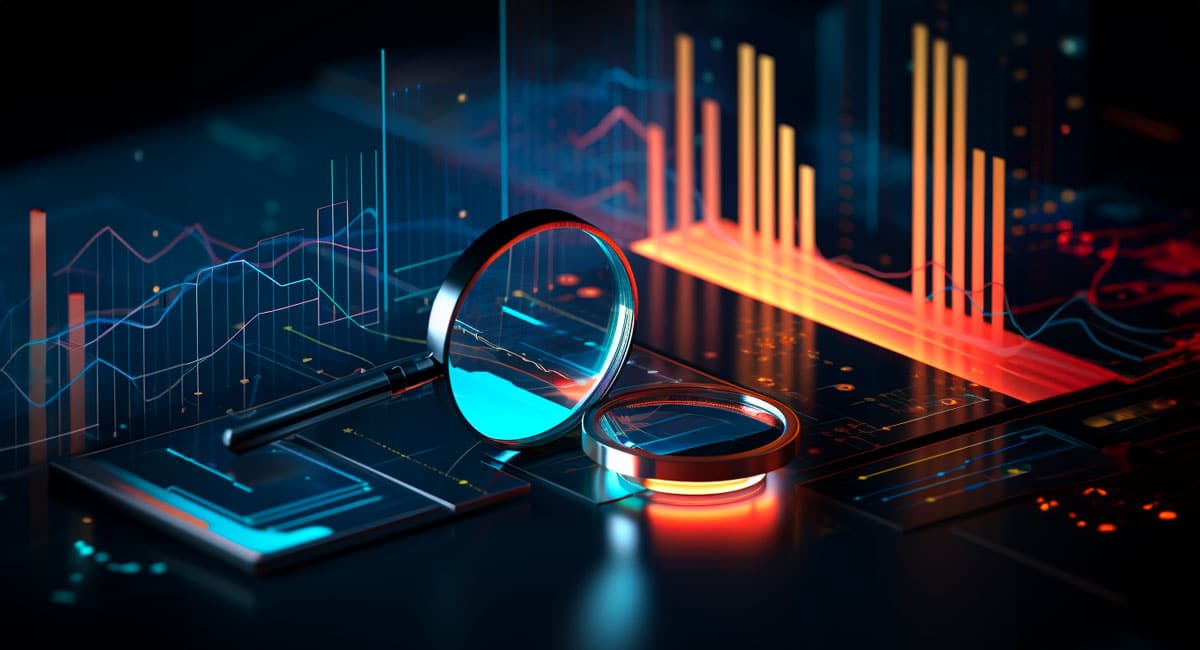
Examples of the difference between Semantic vs. Keyword in Diverse Business Contexts
Let’s explore the nuances between semantic and keyword searches across the following contexts:- A corporate IT Specifier researching knowledge management solutions for their company. This might actually be you right now.
- A Pharmaceutical Industry case related to aspirin and cardiovascular disease
- An Industrial Manufacturing case related to a process engineer’s issues with bottlenecks
A Corporate IT Specifier Example of Semantic versus Keyword Search
Enter the Business Technology Director. She is exploring options to purchase an enterprise knowledge management solution for her company, focusing on how semantic search and generative artificial intelligence can be integrated. She types "enterprise knowledge management with semantic search and generative AI."The lexical keyword approach
The keyword search might yield results that separately discuss 'enterprise knowledge management', 'semantic search', and 'generative AI'. These results could include generic overviews of each technology, general industry articles, or vendor websites mentioning these terms, but not necessarily how they can be integrated or their specific benefits when used together in knowledge management systems.The semantic approach
In contrast, a semantic search might understand the executive's intent to find a synergistic solution combining these technologies. The results could include in-depth analyses of how semantic search enhances knowledge management by understanding context, alongside how generative AI can contribute by creating new content or insights. The semantic search might also provide case studies of successful integrations in similar corporate environments, expert opinions on the future of AI in knowledge management, and specific vendor solutions that offer a combined approach of semantic search and generative AI tailored for enterprise needs. Our specifier is now better empowered to make informed and creative decisions in their choice of technologies, and be a stronger player on their decision committee.Pharmacology Example of Semantic versus Keyword Search
A researcher who wants to understand the connection between aspirin and heart health types "aspirin cardiovascular benefits" into a search engine.The lexical keyword approach
A keyword search would scan through databases and web pages for those exact terms: "aspirin," "cardiovascular," and "benefits." The results might include a wide range of documents mentioning aspirin and cardiovascular topics, but they might not be specifically focused on the benefits of aspirin for cardiovascular health. The researcher could end up with a mix of general information about aspirin, various cardiovascular conditions, and unrelated benefits, requiring them to sift through the results to find relevant information.The semantic approach
In contrast, a semantic search for "aspirin cardiovascular benefits" might understand that the researcher is specifically interested in how aspirin benefits cardiovascular health. The returned results would be more targeted, possibly including clinical study results, pharmacological analyses of aspirin's effect on cardiovascular health, and expert reviews discussing this specific topic. "The semantic search understands that the researcher is seeking the connection between aspirin and its positive effects on cardiovascular conditions, leading to more precise and relevant information. This empowers the consumer to make better health choices and the professional to make more informed recommendations faster."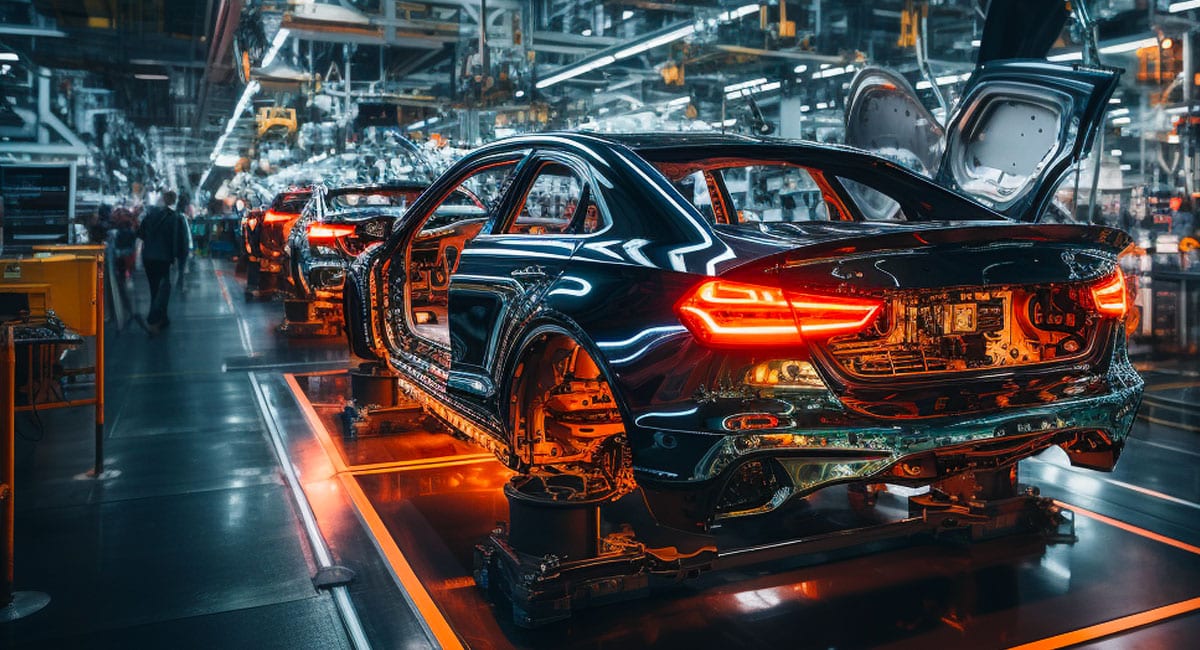
Industrial Manufacturing Example of Semantic versus Keyword Search
Enter, the Industrial Manufacturing Process Engineer. He types "production line bottleneck solutions" into the search field.The lexical keyword approach
The keyword search might return a broad range of results including general articles about production lines, basic bottleneck theory, or generic solutions unrelated to the specific context of the engineer's manufacturing process. The results are based on the presence of the words 'production', 'line', 'bottleneck', and 'solutions' in the content, not necessarily related to the engineer's specific industrial scenario.The semantic approach
In contrast, a semantic search for "production line bottleneck solutions" might understand that the engineer is seeking solutions to improve a manufacturing process. These results might include detailed case studies of similar industrial settings, advanced methodologies specific to the type of production line the engineer is working with, and targeted strategies for identifying and resolving bottlenecks in a manufacturing context. The search understands the context of 'production line bottlenecks' within the framework of industrial process engineering, and will better empower our engineer to identify solutions that ensure uninterrupted throughput of their production line.Key questions to ask for successfully deploying semantic search in your organization
To ensure ROI for your semantic search deployment, there are many success factors to contemplate. These factors include technical infrastructure requirements, data privacy, hygiene, and security, human resource training and change management, content strategy and management, vendor selection, user experience design, analytics, and budgeting. In this section, we will outline critical questions to ask when planning for a successful deployment of semantic search, and provide high-level implications for example industries. You may find this outline helpful for building your own check-list of questions to answer. If you would like help in answering these questions for your business please contact us for assistance.What Infrastructure and Resource Allocation are required for semantic search?
Semantic search can increase demand on your technical infrastructure. Critical questions include the following:- How can we increase server capacity and processing power to manage complex semantic algorithms and data sources?
- What scaling strategies are needed for high-traffic events to support our semantic search system?
- Pharma: Upgrading data centers for processing large-scale clinical trial data.
- Industrial Manufacturing: Expanding server capabilities for real-time product quality monitoring.
- Consumer Retail: Enhancing e-commerce platforms for peak shopping seasons like Black Friday.
What Data Privacy Compliance Measures Should be Considered for Semantic Search?
The more relevant contextual data semantic search can leverage, the more valuable the results delivered. However, additional data sources can increase risk of privacy or security compliance violations. It is important to ask the following:- When using personalized semantic search, what measures are necessary to comply with privacy laws such as the EU’s General Data Protection Regulation (GDPR), the California Consumer Privacy Act (CCPA), South Korea’s General Data Protection Law (LGPD), and others.
- How can we enhance our data collection and storage systems for more compliance across complex data types and sources with varying regulation or permissioning rules?
- Will regulations be managed in a uniform manner across all users of the system, or are there different access privileges required for various roles, work-groups, or individuals, or for exceptional or extraordinary cases?
- Pharma: Implementing advanced encryption for patient data in research databases.
- Industrial Manufacturing: Securing proprietary manufacturing data while allowing semantic analysis.
- Consumer Retail: Integrating GDPR-compliant practices in customer data handling for personalized searches.
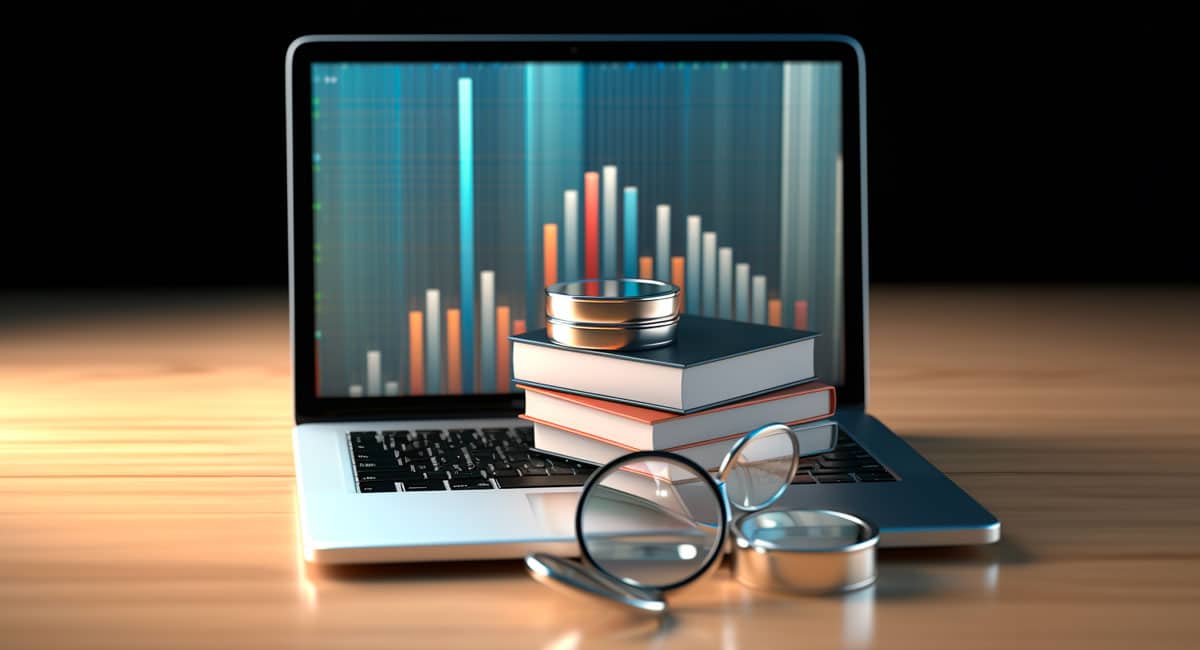
What Employee Training and Skill Development is Required for Deploying Semantic Search?
Far too many organizations neglect the human side of technology transformation and value realization. Let’s contemplate.- What training is needed for our IT staff to manage and troubleshoot advanced semantic search technologies?
- How can we upskill content creators, marketers and data managers to optimize content and data for semantic search? Will we need a formal change management program, or will training be enough?
- How can we encourage user experience behaviors to realize the benefits of semantic search?
- Pharma: Training bioinformatics teams in semantic data analysis for drug discovery.
- Industrial Manufacturing: Educating engineers on semantic technologies for predictive maintenance.
- Consumer Retail: Training marketing teams in semantic SEO and content optimization.
Integration with Existing or Potential Systems
Interoperability is the name of the game. It is absolutely essential to consider the integration of semantic search with other technologies that extend its power.- How can we ensure compatibility between our semantic search tools and existing databases or content management systems?
- Are there any specific hardware or software requirements for deploying these technologies?
- Do we need to upgrade our existing systems to ensure compatibility and optimal performance?
- What technologies should we integrate to extend the power of intent-driven search, such as Retrieval-Augmented Generation (RAG).
- What additional technologies and interfaces should we consider to support operation, presentation, and interaction with content beyond just search results pages? E.g., map interfaces, data visualization and manipulation, configurators, editors, or other tools?
- Which integrations should we prioritize on our roadmap?
- Pharma: Ensuring compatibility of semantic search tools with drug discovery databases.
- Industrial Manufacturing: Integrating semantic search into legacy supply chain management systems.
- Consumer Retail: Merging semantic search capabilities with existing online and offline inventory systems.
Content Strategy and Management
The quality of content and data is critical to the functionality and value that semantic search can deliver. Here are key questions to consider.- What types of content will be indexed and made searchable? (e.g., internal documents, product databases, external web content)
- How comprehensive does our corpus need to be to ensure effective retrieval and generation?
- How comprehensive and clean does our content or data need to be to match the domain model required for optimal machine learning?
- How Will Content Be Maintained and Updated? Especially in light of the future needs of semantic search and AI augmented operations and creativity?
- What processes will be in place for updating and maintaining the indexed content?
- How will we ensure the content remains relevant, accurate, and up-to-date?
- What are the Data Sources and Their Quality? Do we have documented quality management processes in place, what are the parameters, and are they consistently represented across the content or not?
- How do we assess and ensure the quality and reliability of these sources?
- Pharma: Ensuring rules-based compliance firewalls between commercial and medical affairs for regulated information and interactions in some cases but less so for less regulated information types and interactions.
- Industrial Manufacturing: Developing technical content strategies for enhanced product discoverability and variant configurability based on combinations of ingredient products.
- Consumer Retail: Crafting product descriptions, modularized marketing content, and targeted metadata optimized for semantic searches that can drive personalized product recommendations, catalogs, and support documentation based on intent and customer profile.
Analytics and Continuous Improvement
How can we implement analytics to monitor and continually refine the effectiveness of our semantic search? Example implications:- Pharma: Using analytics to refine semantic search in drug research databases.
- Industrial Manufacturing: Continuously improving semantic search for efficient production processes.
- Consumer Retail: Leveraging analytics to understand customer search patterns and refine the search experience.
Cost Implications and Budgeting
How should we budget for the initial setup, ongoing maintenance, and upgrades of semantic search systems? Example implications:- Pharma: Working across commercial and medical affairs to identify budget sharing responsibilities for implementing CRM driven next best actions that use semantic search to provide personalized content to drive next best actions, when content responsibilities and benefits span multiple reporting and budgetary lines.
- Industrial Manufacturing: Allocating funds for integrating semantic search into production systems.
- Consumer Retail: Planning expenditures for semantic search integration across e-commerce, physical stores, dedicated mobile apps; and to ensure leverage of data across multiple systems such as purchase history, loyalty programs, or other sources that reveal intent and indicate purchase propensities.
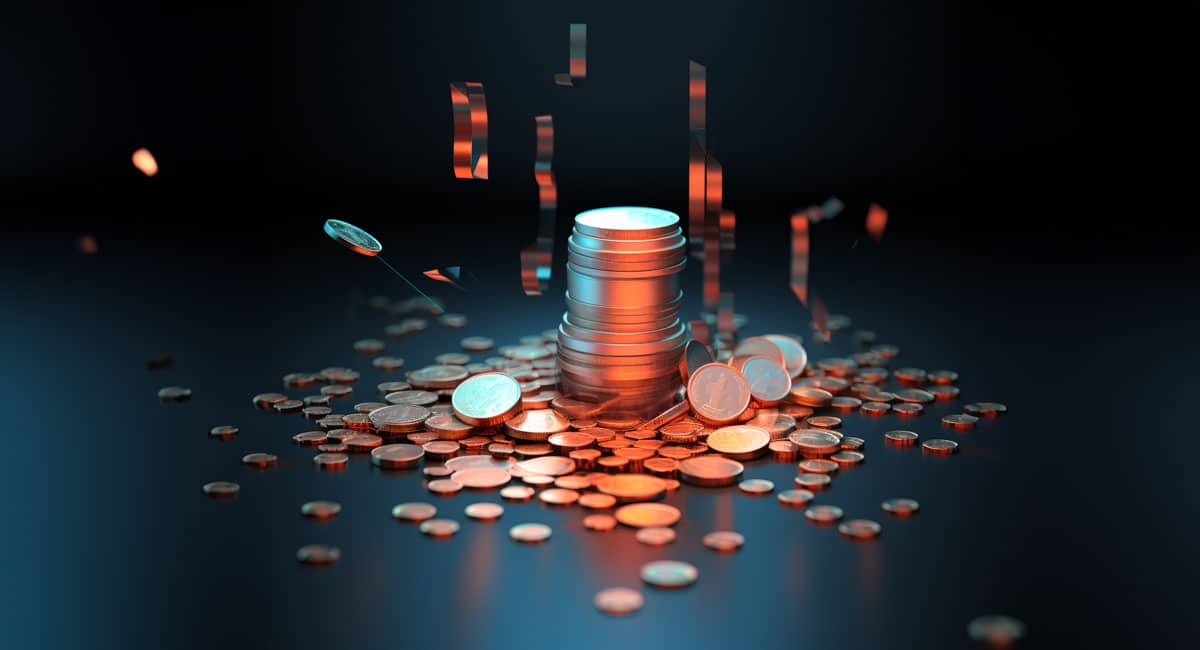
Vendor Selection and Partnership
How do we choose the right technology partners for our semantic search solutions?- Pharma: Choosing vendors with expertise in regulatory requirements for pharmaceutical companies and who understand how to integrate with major industry-specific vendors, even if there are challenges in collaboration.
- Industrial Manufacturing: Selecting partners proficient in:
- Addressing complex variation in requirements for very similar searches
- Understanding complex channel dynamics such as 3rd party system integrations or variant content display depending on user channel preferences or vendor-to-channel agreements that affect what should be displayed to one user versus another for the same search.
- Consumer Retail: Collaborating with vendors that offer consumer-focused semantic search solutions for retail.