Generative artificial intelligence (GenAI) has emerged as a powerful tool for content creation, but often requires a human in the loop to ensure the outputs are valuable. The value of GenAI includes productivity gains, opportunity for revenue growth, and better accuracy for your organization’s content, but human involvement remains essential. In this blog we look at examples of GenAI’s benefits and the need for a human in the loop.
What is Human in the Loop?
Human in the Loop (HITL) is one of the easier terms to understand in the glossary of AI terminology. A perfectly efficient automated process is one that doesn’t need to be monitored — such as a coffee machine set to pour exactly 8 ounces of coffee. Many machines can perfectly execute these kinds of simple tasks. As artificial intelligence is used for more complex tasks, it is likely the technology will make an error.
These errors aren’t so monumental to negate the productivity gained from relying on AI. Most of the time these errors can be resolved with a member of your workforce checking the AI’s outputs. Assigning a person to oversee the outputs of an AI is what we call a human in the loop. Acting as a HITL doesn’t require any technical understanding of AI. As the technology of AI advances and trust in their outputs increase, HITLs will no longer be necessary.
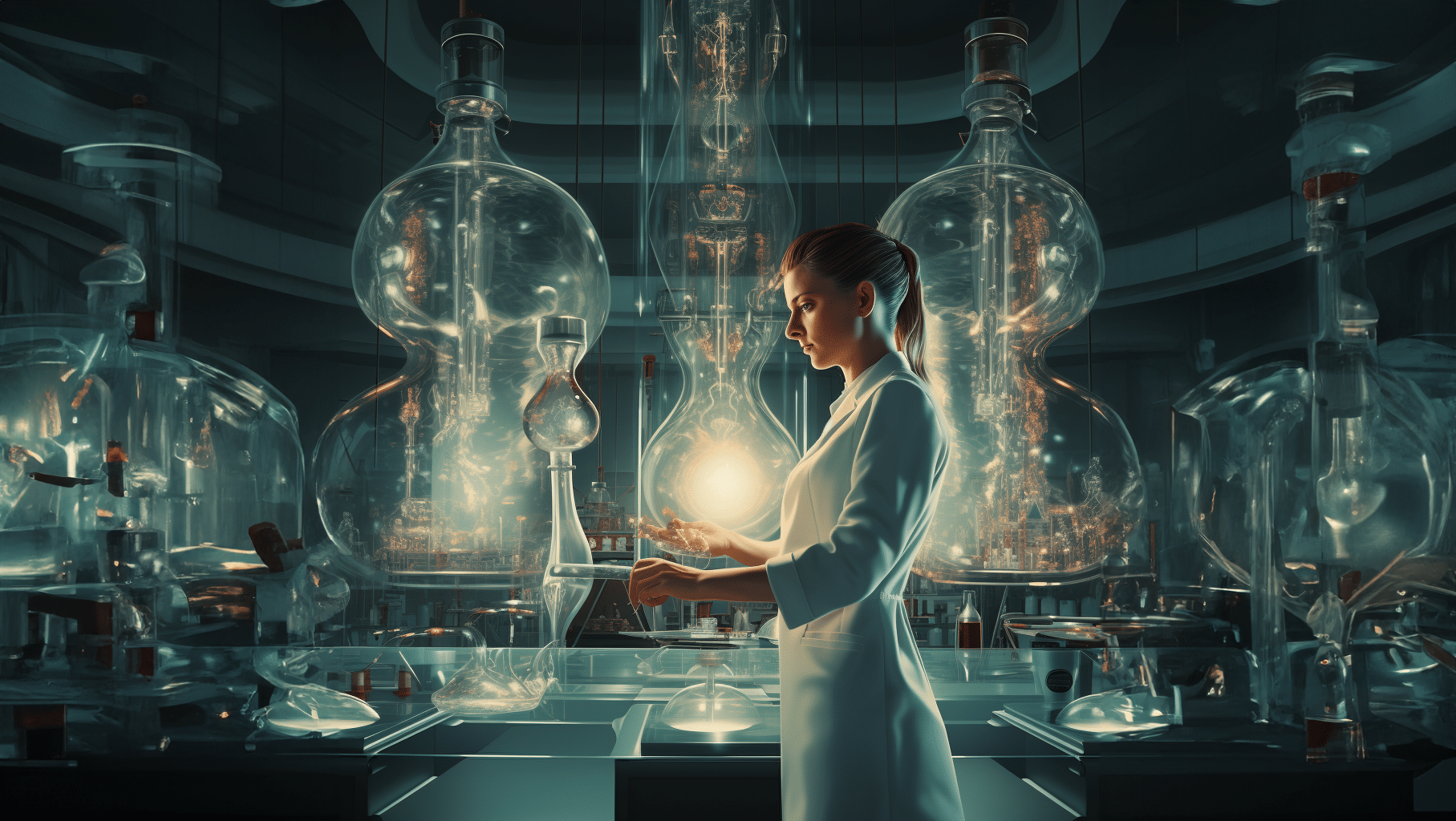
Generative AI is Everywhere
Generative AI is a broad term that applies to any content output by an AI. Most people are familiar with Chat-GPT and other large language models that produce text-based outputs, but it’s not exclusive to text. Image generators such as DALL-E or Midjourney can produce images from simple text prompt. Adobe announced a suite of GenAI tools for their products that can generate video content in addition to text and images. The availability of these tools has made it possible for everyone to generate some version of text, image, or video content.
The proliferation of GenAI content has influenced a variety of industries. Websites and blogs are heavily reliant on search engine optimization standards — which include promoting pages that contain original image content. This barrier for results considered higher quality has been shattered by the ease of creating GenAI images. This is also true for written content. Businesses and executives don’t need a dedicated writer to generate content, but can instead rely on GenAI drafts. This has made the labor-intensive process of written content significantly easier.
The greater availability of content generated by AI has been followed by higher standards for these types of assets. Audiences can easily recognize when content has been generated by AI. These indicators aren’t necessarily “errors,” but they prompt the need for humans in the loop.
The Crucial Role of Human Insight
Generative AI has revolutionized content generation, but the content made by GenAI often includes clear indicators of its origin. A popular tweet sharing images generated by Midjourney was criticized for depicting people with seven fingers or seemingly more than 40 teeth. At first glance the images appear to depict lifelike ultra realism, but human insight reveals errors not picked up by the GenAI tool.
Generative AI tools are heavily reliant on the inputs from their users. A human may have an intuitive grasp of an instruction, but an AI may make creative decisions that depart from these inputs. An image GenAI tool may carry associations for words and their typical usage. For example, asking Midjourney to create an image of “robots floating around an office space” can result in the office space being depicted underwater since “float” is highly correlated with water. This is the type of error a human in the loop can resolve.
Visual tools such as Midjourney and DALL-E provide visceral examples of the need for a human in the loop, but this is true for all generative tools. The context provided by your input may make assumptions on the AI’s knowledge that may not be there. For example, if you provide a page from a novel and request the GenAI to write a chapter based on that novel — it doesn’t have any knowledge of the proposed novel outside of the single page. If a character isn’t immediately referenced on the provided page, then the AI cannot incorporate that information in its output.
This example can be extrapolated to errors derived from prompts requesting the GenAI to elaborate on incomplete datasets — such as analyzing market conditions or risk assessment based on a limited pool of data. For example, requesting an insurance risk assessment of a new region without specifics may result in the AI generalizing assumptions. If you ask for an assessment on a region in the northwest of the United States, it might assume the average density of forested areas and the risk of uncontrolled fires. This could be helpful if the region you selected is typical, but only a human insight could catch if this assumption is accurate or misapplied.
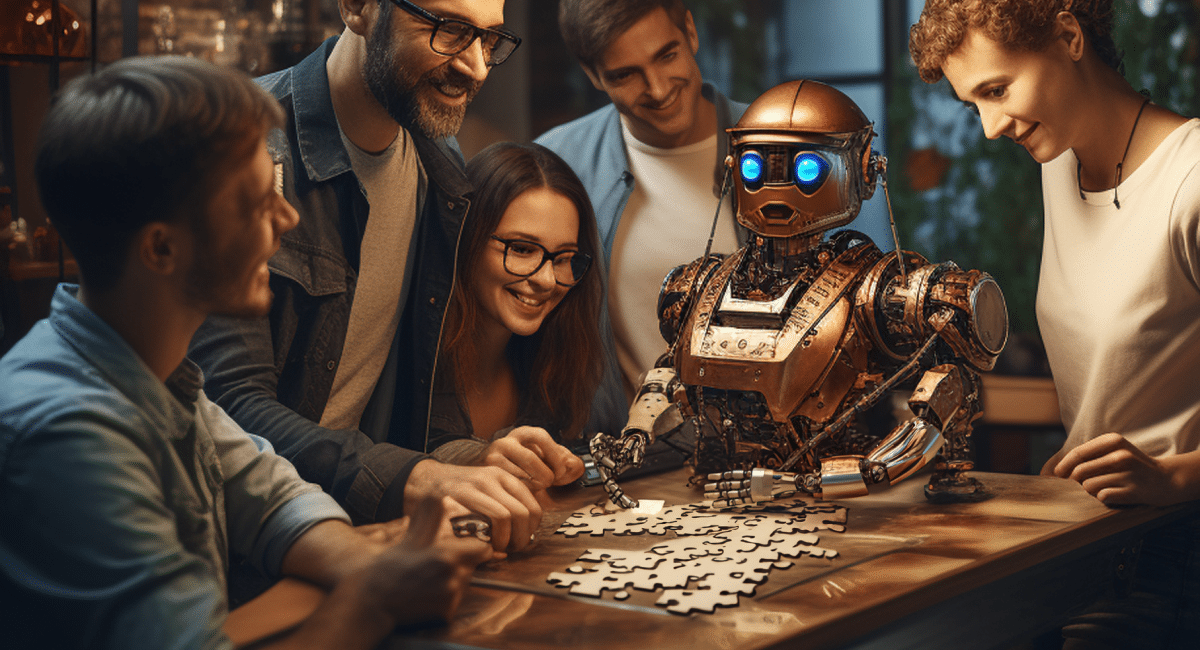
Human in the Loop Makes GenAI Better
The necessity of human in the loop may appear to be a limitation of GenAI, but most generated content – AI or otherwise — requires personalization. Email marketing, advertising, and customer service are all industries that benefit from the productivity gains of GenAI content but will always perform better when the output is personalized to the intended audience.
Other AI solutions expedite laborious processes to accelerate a human’s involvement in an otherwise repetitive task. For example, GenAI tools used in medical or legal research allow users to narrow the scope of papers or cases to review for future innovation. The human in the loop can aggressively eliminate potential information sources curated by the AI and focus on what’s most valuable for their goals.
Alternative to Human in the Loop: Augmenting GenAI with Your Content
One method of checking content provided by GenAI is to augment its outputs with your knowledge base. Mapping a GenAI solution to your organization’s knowledge ensures the standards and requirements of your organization are applied to the GenAI’s outputs. Domain-specific generated content results in greater operational efficiencies. It also prevents the generalist perspective common in LLMs and other GenAI tools. Providing your organization’s proprietary data and perspective aligns the GenAI’s outputs to match your organization’s goals and objectives.
Shelf specializes in preparing your organization’s knowledge for GenAI tools. Our knowledge management platform ensures your organization’s content becomes an asset for your AI strategy. If you’re not certain where to start with integrating AI into your business, chat with our team for easy first steps to assess your organization’s readiness for AI.
Human in the Loop Validates Outputs and Applies Quality Assurance
The proliferation of GenAI has made content generation more accessible and efficient for everyone, but it still requires an expert to oversee its outputs. A human in the loop can validate outputs and apply quality assurance — especially in domains where specialized knowledge and expertise are paramount such as medical or legal research. While the future of AI promises of a fully automated society, the immediate use cases of this technology require a human in the loop to ensure the best outcome. Leveraging the strength of GenAI along with a dedicated role for overseeing outputs ensures high-quality, relevant, and impactful content.