Generative AI is changing the way businesses operate, helping them work faster, smarter, and more creatively. From automating tasks to delivering personalized customer experiences, it’s giving companies a real edge in today’s competitive landscape.
Let’s dive into how GenAI is transforming enterprises and the key ways it can benefit your business.
What is Generative AI?
Generative AI is a type of artificial intelligence that creates new content, whether it’s text, images, or even code. Unlike traditional AI, which relies on predefined patterns or models to make decisions, generative AI uses machine learning algorithms to produce fresh, original outputs based on input data. This makes it a powerful tool for creating personalized content at scale.
You can think of generative AI as the engine behind chatbots, image generators, and even tools that help write code. It’s all about generating something new from existing information, and it’s transforming how enterprises can handle content creation, automate processes, and enhance customer experiences.
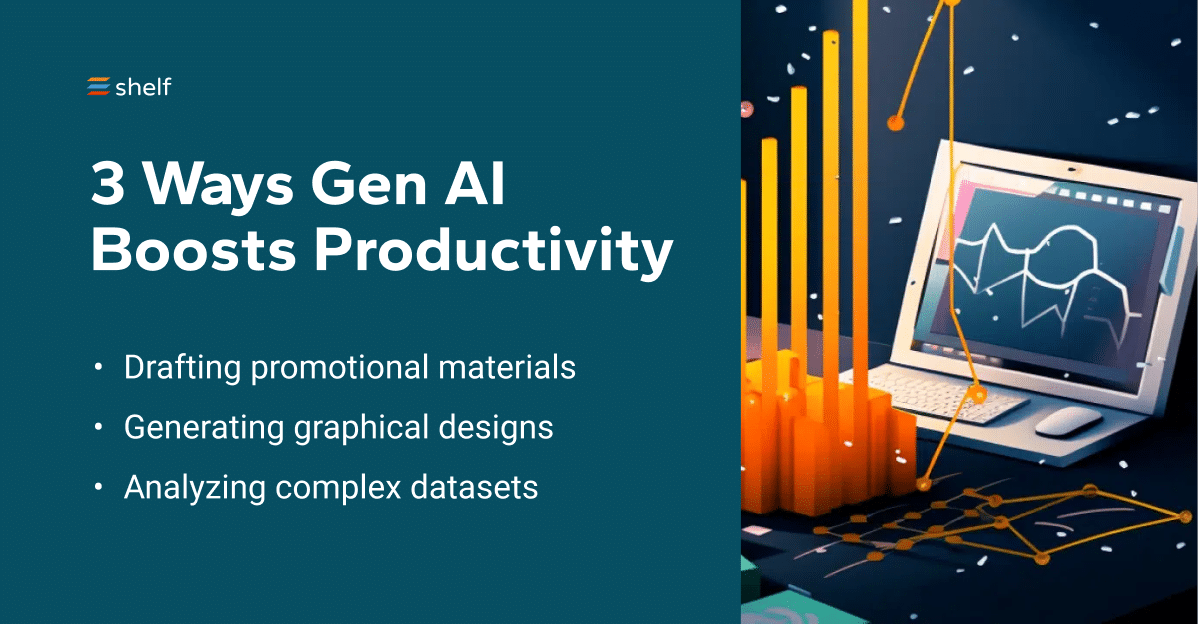
How GenAI Works: The Basics
Generative AI works by training on large datasets and learning to understand patterns. It uses these patterns to predict what should come next when given a prompt. For example, if you ask a generative model to write a product description, it draws from the data it’s learned, like past product descriptions, and generates one that fits the context.
Here’s a simplified breakdown of how it works:
- Training: The model is fed a large amount of data, teaching it to recognize patterns in language, images, or other data types.
- Prompt: You give it a starting point—a text prompt, an image, or another form of input.
- Generation: The model uses what it’s learned to create something new, whether it’s a written response, a generated image, or an algorithm.
Generative AI vs. Predictive AI
While both generative AI and predictive AI rely on data, they serve very different purposes. Predictive AI focuses on forecasting future outcomes based on historical data. It’s great for tasks like predicting customer churn or forecasting demand.
Generative AI, on the other hand, creates new content. While predictive AI might tell you which customers are likely to cancel their subscription, generative AI can craft personalized messages to re-engage them.
In short, predictive AI helps you see what might happen next, while generative AI helps you create what comes next.
Generative AI Terms and Definitions
Understanding key terms related to generative AI helps you grasp how these technologies work and their impact on your organization.
- Natural Language Processing (NLP): NLP refers to the branch of AI that enables machines to understand, interpret, and generate human language.
- Transformer: A transformer is a neural network architecture that revolutionized AI by improving how models process sequential data. It led to advancements in tasks like language translation and text generation.
- Large Language Model (LLM): An LLM is a type of AI model, like OpenAI’s GPT, trained on vast amounts of text data to understand and generate human-like language. It excels at generating coherent text, answering questions, and summarizing information.
- Pre-trained Model / Foundation Model: A pre-trained model (or foundational model) is trained on massive datasets before being fine-tuned for specific tasks. These models provide a starting point, saving time and resources by leveraging prior learning.
- Fine-tuning: Fine-tuning is the process of taking a pre-trained model and adapting it to a specific task or dataset, such as the particular needs of your business.
- Hallucination: In generative AI, a hallucination occurs when the model generates false or nonsensical information that is not based on the input data or reality.
- Multi-modal AI: Multi-modal AI can process and generate different types of data simultaneously, such as text, images, and audio. This makes it powerful for applications that require a blend of data types, like image captioning or video analysis.
- Retrieval Model: A retrieval model is designed to retrieve relevant information from a large dataset in response to a query, often used to enhance generative AI by providing accurate, contextually relevant data.
- Vector Store: A vector store is a database that stores data in vector format. It’s used in AI to match and retrieve similar data points efficiently, such as documents or images based on their features.
- Enterprise AI: Enterprise AI refers to AI applications designed specifically for businesses, focusing on automation, decision-making, and scaling processes.
Why is GenAI Important for Enterprise?
Generative AI is a game-changer for enterprises because it unlocks new ways to automate tasks, personalize experiences, and otherwise drive business value. There are countless ways you can use it to streamline operations, enhance customer engagement, and innovate faster than ever before.
Automating Content Creation
Generative AI allows you to automate repetitive content creation tasks, from writing reports to drafting marketing copy. This means you can free up valuable human resources for more strategic work, while maintaining a consistent output of high-quality content.
Enhancing Customer Experiences
GenAI enables you to deliver personalized customer interactions at scale. Whether it’s generating custom product recommendations or responding to customer queries in real time, AI can tailor experiences to each individual.
Improving Decision-Making
With GenAI, you can generate actionable insights from vast amounts of data. The AI can help you quickly summarize information, identify trends, or create forecasts, allowing your team to make faster, more informed decisions.
Scaling Business Processes
As enterprises grow, so does the complexity of their operations. GenAI helps you scale these processes by automating tasks such as document generation, data analysis, and even customer support. This enables you to expand without overburdening your workforce.
Fostering Innovation
Generative AI opens the door to new innovations, like automating code generation or designing new products. GenAI can help your enterprise stay ahead of the competition and continuously evolve.
Benefit of Generative AI for Enterprise
Generative AI is changing the game for business leaders, offering a range of benefits that can make your operations smoother, faster, and more competitive. Here’s a closer look at how it can help:
1. Boost Efficiency with Automation
Generative AI can handle the time-consuming, repetitive tasks like writing reports, drafting marketing materials, or responding to customer queries. By letting AI take care of these, you free up your team to focus on bigger, more strategic projects.
2. Personalized Customer Interactions—At Scale
You can use GenAI to deliver highly personalized experiences for your customers without stretching your team thin. Whether it’s creating tailored emails or recommending products based on individual preferences, AI helps you engage each customer in a way that feels personal and meaningful, which can lead to better satisfaction and loyalty.
3. Better Data Analysis and Decision-Making
Generative AI can quickly sift through massive datasets and pull out trends, insights, or summaries. It’s like having an extra set of eyes on your data, helping you make smarter decisions faster and stay ahead of changes in the market.
4. Cut Costs by Optimizing Processes
With AI streamlining workflows and automating various tasks, you can reduce operational costs. Whether it’s cutting down the need for manual content creation or speeding up development cycles, GenAI helps you use your resources more efficiently and save money in the long run.
5. Fuel Innovation and Creativity
AI isn’t just for crunching numbers; it’s also a creative tool. From helping design new products to assisting with code generation, GenAI opens up new possibilities for innovation. For example, it can generate multiple prototypes in minutes, helping your team refine ideas and bring products to market faster.
6. Smarter Enterprise Search
GenAI takes enterprise search to the next level. It helps you quickly find relevant information from massive datasets, even if the data is messy or unstructured. This improves knowledge management and speeds up decision-making across the board.
7. Scalable Solutions for Growing Businesses
As your business grows, so do your data and operational needs. GenAI scales easily, which lets you handle more data, more customers, and more complexity without putting extra strain on your team or infrastructure. It helps ensure that you can grow smoothly while staying efficient.
Limitations of Generative AI for Enterprise
While GenAI offers many advantages, there are some key challenges to keep in mind:
- Data Quality: Generative AI relies on clean, unbiased data. If your data is flawed, the AI will generate unreliable or misleading outputs.
- Hallucinations: AI can sometimes produce factually incorrect or nonsensical content, especially if not closely monitored.
- Context Limitations: AI may not fully grasp the specific context or nuances of your business, leading to off-target results.
- High Costs: Running large AI models requires significant computing power, which can be costly for smaller businesses.
- Legal and Ethical Considerations: AI can introduce issues like biased outputs, privacy risks, and potential copyright violations.
- Integration Challenges: Connecting AI models to legacy systems, databases, or business platforms can be complex and time-consuming.
- Lack of Transparency: AI models often operate as “black boxes,” making it difficult to explain how decisions or outputs are generated.
- Continuous Maintenance: AI models require regular updates, fine-tuning, and retraining to remain effective as your data and business evolve.
By understanding these limitations, you can better prepare for successful AI implementation in your enterprise.
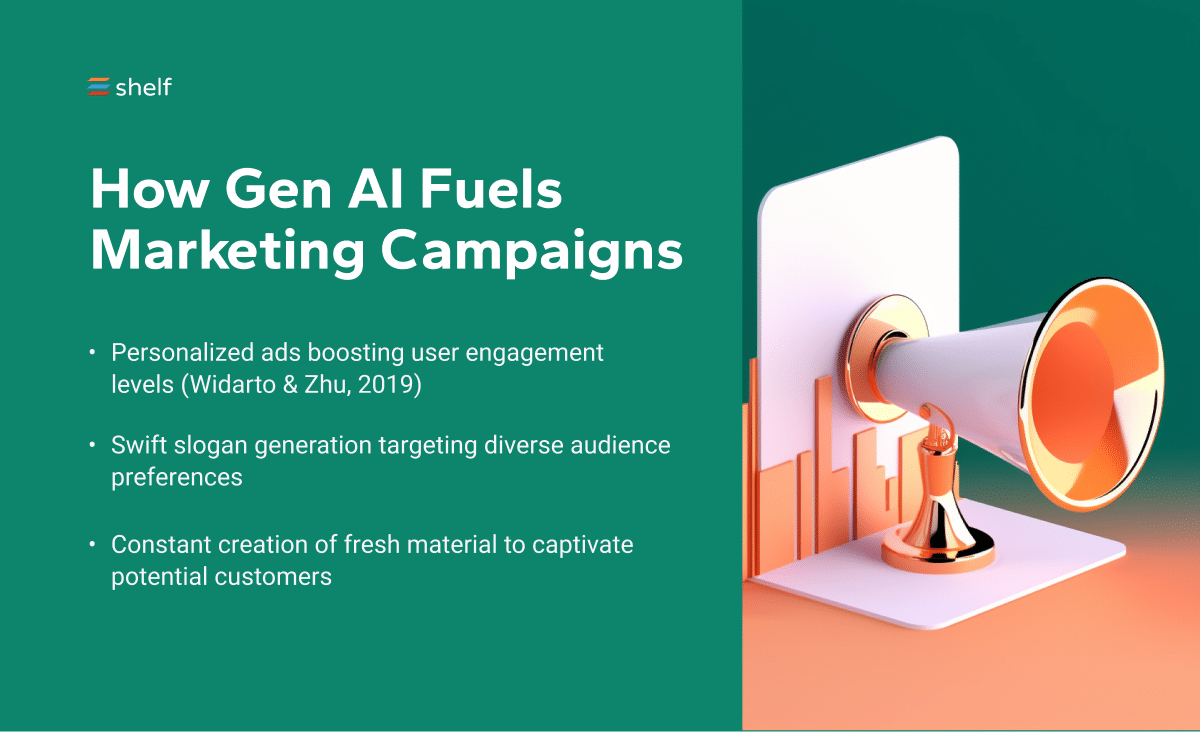
Enterprise GenAI Use Cases
Generative AI is shaking things up for business models across industries. Here’s a look at some of the most impactful ways GenAI is making a difference:
1. Automated Content Creation
Tasks like writing social media posts, email newsletters, product descriptions, or other forms of content can be created with consistent, personalized content without the manual effort. GenAI can also handle internal reports or technical docs, speeding up the process and ensuring accuracy.
2. Customer Support Automation
With GenAI, you can provide around-the-clock customer support without the need for human agents. AI-powered chatbots handle customer interactions and offer instant responses to customer inquiries based on historical data and common queries. AI can even draft and send personalized emails.
3. Enterprise Search and Knowledge Management
AI-powered search tools make it easier for employees to pull relevant information from internal databases, documents, or communication tools. AI can help with knowledge management by summarizing and organizing documents, improving how teams share and access information.
Shelf’s suite of AI-enabled knowledge management tools gives you unparalleled transparency into the quality of your data, provides governance that enables continuous improvement, and uses automation to deliver better answers everywhere you need them.
4. Data Synthesis and Simulation
GenAI creates synthetic data to simulate real-world scenarios, ideal for testing, research, and development, which is helpful when actual data is limited or sensitive. It can also create training simulations in industries like healthcare and finance, giving employees risk-free environments to practice decision-making.
5. Personalized Marketing and Sales
AI helps you deliver more personalized experiences to customers. By analyzing customer data, AI can suggest personalized products that boost engagement and conversions. It can also generate customized ad copy for different customer segments and even help you create innovative products.
6. Financial Forecasting and Analysis
AI helps businesses make smarter financial decisions by analyzing large datasets, giving you real-time insights into your business’s financial health. GenAI can forecast trends based on historical data, helping you manage risk and make informed decisions.
7. HR and Recruitment Automation
AI can streamline the hiring process and improve employee engagement by screening resumes and matching candidates to job openings. It can also analyze employee surveys which helps HR identify trends and areas for improvement.
8. Healthcare Innovations
In the healthcare industry, GenAI assists with research, diagnostics, and patient care. It can summarize patient records, lab results, and research papers to support better decision-making for healthcare professionals. It can even generate molecular structures for potential drugs, speeding up the discovery process and cutting costs.
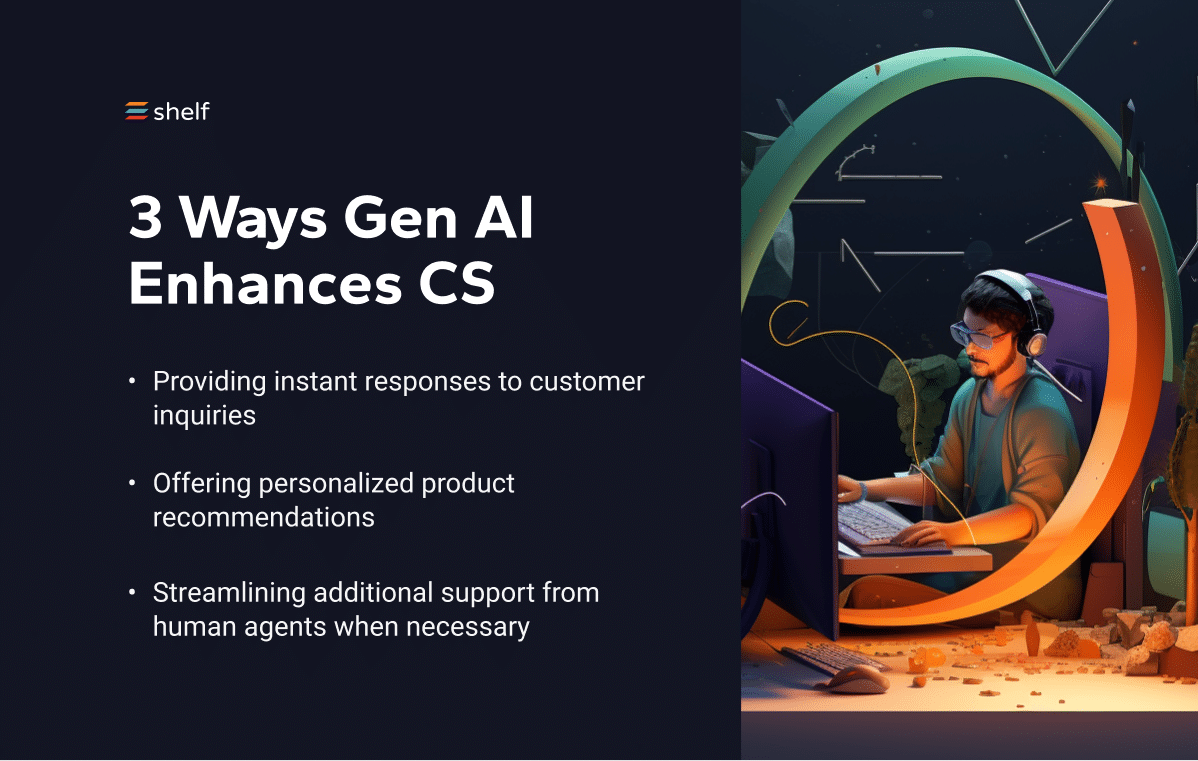
Where to Start with Enterprise Generative AI
Implementing generative AI in your enterprise can seem daunting, but starting with a clear strategy will help ensure success. Here’s a guide on where you should begin when adopting GenAI in your organization:
1. Identify Use Cases with High Impact
The first step is to identify where generative AI can make the most significant impact. Start by evaluating your current challenges and processes that could benefit from automation or optimization.
Look for repetitive tasks: Areas like content creation, customer service, and report generation are prime candidates for GenAI.
Focus on data-heavy processes: If your enterprise deals with large volumes of data, such as in financial analysis or product design, generative AI can help you unlock insights and streamline workflows.
Target areas for innovation: Think about where GenAI can help you create new products, optimize services, or improve customer experiences.
2. Assess Data Readiness
Generative AI models are highly dependent on quality data. Before diving in, you should assess whether your data is clean, well-organized, and sufficient for training AI models.
Centralize your data: Ensure that your enterprise data is easily accessible and well-structured. You may need to consolidate data from various silos into a central repository.
Improve data quality: Clean and enrich your data to minimize errors and bias. Remember, better data leads to better AI outputs.
Prepare unstructured data: If your data includes unstructured content (like emails, documents, or images), you’ll need to organize and categorize it for GenAI to process effectively. Tools like Shelf’s unstructured data management platform can make your data GenAI-ready.
3. Start with Pre-trained Models
Pre-trained models, or foundational models, provide a great starting point for enterprises new to AI. These models are trained on large datasets and can be fine-tuned for specific tasks with minimal resources.
Choose a pre-trained model: Select an AI model that aligns with your goals, whether it’s a large language model (LLM) for content generation or a transformer for processing complex data.
Fine-tune for specific use cases: Adapt the model to your business’s unique needs by feeding it domain-specific data. This step ensures the AI generates outputs relevant to your industry.
4. Build a Cross-Functional Team
Successful GenAI implementation requires collaboration across departments. You’ll need to build a team with diverse skills to manage different aspects of the AI journey.
Include data scientists and AI specialists: These experts will help develop, train, and fine-tune AI models to meet your enterprise’s needs.
Involve business leaders: Stakeholders from marketing, customer service, and operations should be involved to ensure the AI solution addresses real business challenges.
Ensure IT support: You’ll need IT to handle integration with existing systems and ensure that your infrastructure can support GenAI applications.
5. Pilot a Small Project
Rather than overhauling entire processes, start small with a pilot project. This allows you to test how well generative AI integrates into your enterprise and provides an opportunity to refine your approach before scaling up.
Select a low-risk use case: Choose an area where AI can make a tangible impact without disrupting critical business operations, such as automating internal reports or enhancing customer support chatbots.
Measure results: Track key performance indicators (KPIs) like time savings, customer satisfaction, or cost reductions to evaluate the effectiveness of your AI implementation.
6. Ensure Governance and Ethical Compliance
As you begin using generative AI, establish strong governance to manage the ethical, legal, and operational challenges that come with it.
Set up data governance: Ensure your AI is using compliant, secure, and high-quality data. This helps avoid data privacy violations and ensures the AI generates trustworthy outputs.
Develop guidelines for responsible AI use: Establish ethical standards to avoid issues like bias or misinformation in AI-generated content.
Implement monitoring and auditing: Continuously monitor AI outputs to catch hallucinations or inaccuracies and ensure the model remains aligned with your business objectives.
7. Scale and Optimize
Once your pilot project proves successful, you can start expanding your AI initiatives to other areas of the business. Ensure you are continuously optimizing models as new data becomes available or as business needs evolve.
Expand to new use cases: After a successful pilot, roll out AI in other departments, such as marketing, sales, HR, or product development.
Continuously update models: Fine-tune models regularly to keep up with changing business demands and evolving datasets.
Invest in AI infrastructure: As your AI use cases grow, make sure your infrastructure can scale, whether through cloud services or on-premises solutions.
What’s Next for Enterprise Generative AI?
Generative AI is still in its early stages, but the future looks promising for enterprises that embrace it. As AI models become more sophisticated, you can expect even greater opportunities for automation, personalization, and innovation across all business functions. The key will be staying agile and adapting to the rapid pace of AI advancements.
Here’s what you can anticipate moving forward:
- Generative AI tools will continue to evolve, offering deeper contextual understanding and more accurate outputs. This means you’ll see fewer issues with hallucinations and more reliable AI-generated content.
- As AI technology matures, it will become increasingly embedded across departments—from HR to finance, marketing, and product development.
- With more enterprises adopting AI, there will be a stronger focus on governance, ethical usage, and model transparency.
- Generative AI will open up new frontiers for innovation, from hyper-personalized customer experiences to advanced product design.
In short, generative AI is set to reshape how you operate, compete, and grow. By investing in GenAI now, you position your enterprise to take full advantage of its potential, staying ahead of the curve as the technology continues to evolve. The winning edge lies in how well you integrate, scale, and optimize these tools for future success.