Artificial intelligence engines need data to learn and operate, but the data you and I find meaningful is foreign to machines. Machines need data translated to their preferred language: math. This conversion happens with the help of vectors.
In this guide, we’ll tell you everything you need to know about vectors in machine learning, including how they work, their operations, and their role in AI.
What are Vectors in Machine Learning?
Vectors are mathematical representations of data that have both magnitude and direction. We use them to convert data to math that machines can understand, process, and analyze.
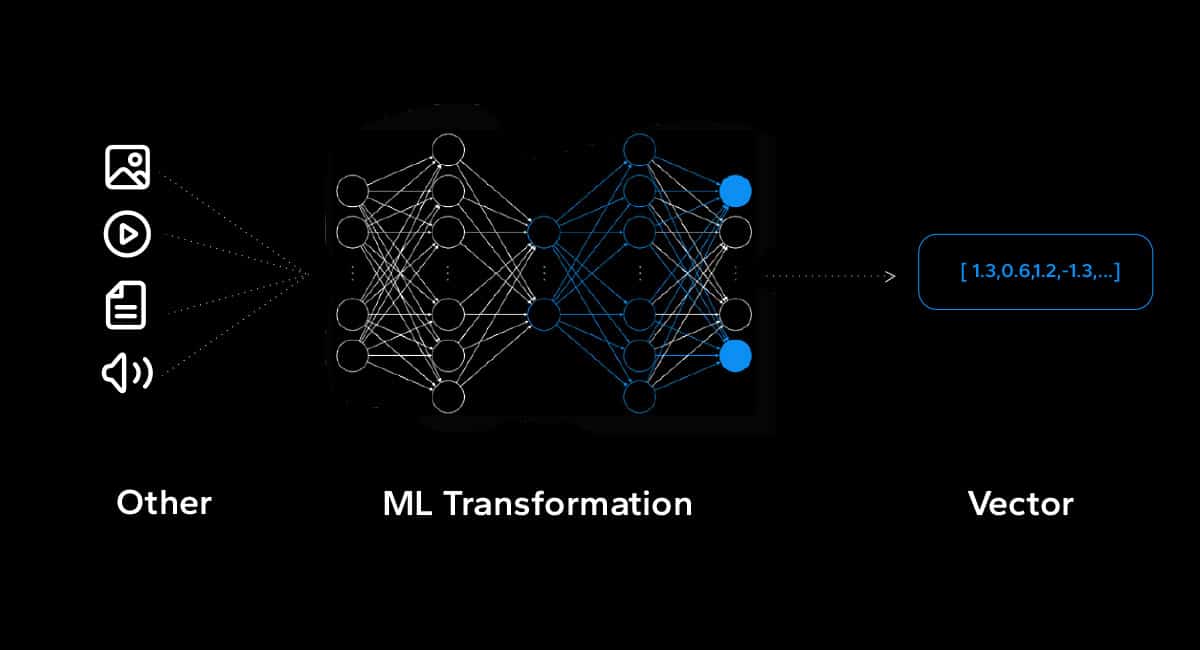
Think of vectors as arrows that represent things with both a magnitude and a direction. The length of the arrow, often referred to as magnitude, represents the size or value associated with the vector. The direction of the arrow indicates the orientation or position in space.
Vectors help us describe quantities like displacement, velocity, and force. By using vectors, we can easily understand and analyze relationships between different things and make predictions.
Vectors in machine learning provide a powerful way to visualize and understand relationships in two-dimensional and three-dimensional spaces. They help us work with data that has multiple dimensions.
In fields like machine learning and data science, vectors are used to represent features and data points. They help transform real-world data into structured mathematical objects. Then, computers can use their language – math – to calculate quantities, compare values, and create mathematical models.
Vectors in Two-Dimensional and Three-Dimensional Spaces
In two dimensions, vectors are used to represent quantities related to flat surfaces. They enable us to describe movements, positions, velocities, and forces in a plane.
In three dimensions, vectors help us understand objects and phenomena in space. They allow us to describe position, motion, and forces acting in three mutually perpendicular directions. This is particularly important when dealing with complex systems involving multiple variables.
The Cartesian Coordinate System
The Cartesian coordinate system is a convenient way to represent vectors both visually and numerically. It consists of perpendicular axes (usually labeled as x, y, and z) that intersect at a common origin.
In the Cartesian coordinate system, a vector can be represented as an ordered set of values along each axis. For example, in two-dimensional space, a vector is represented as (x, y), where x represents movement along the x-axis and y represents movement along the y-axis. In three-dimensional space, the vector is represented as (x, y, z), where z accounts for the movement along the additional z-axis.
This representation allows us to locate points and describe directions and movements precisely, which provides insights into their magnitude, direction, and relationships with other vectors or points in space.
Types and Properties of Vectors
Vectors come in several types, each serving a specific purpose in representing and analyzing quantities.
- A unit vector is a vector with a magnitude of 1. It represents direction without any scaling effect. They often indicate a specific direction or orientation.
- The zero vector has a magnitude of 0. It represents a point in space without any displacement or quantity associated with it. It often references a point or origin.
- Position vectors represent the location or position of a point relative to a reference point or origin. They describe the displacement from the origin to the desired point.
- Resultant vectors arise from the addition or combination of two or more vectors. They represent the overall effect or outcome of combining multiple vector quantities.
- Equal vectors have the same magnitude and direction. They represent different instances of the same vector quantity.
Vectors are commonly represented using specific notation and symbols to distinguish them from other mathematical entities. They are usually denoted using boldface letters in written form, such as v or a, to differentiate them from scalars or other variables.
The magnitude of a vector is represented using vertical bars or double vertical bars. For example, |v| or ||v|| represents the magnitude of vector v.
In some cases, vectors may be denoted using subscripts or arrows. For example, v₁, v₂, v₃ or →v to represent different components or to emphasize the vector nature.
Scalars and Their Relationship with Vectors
Scalars are mathematical quantities that possess magnitude but do not have a specific direction associated with them. They represent values like temperature (e.g., 30 degrees Celsius), time (e.g., 5 minutes), mass (e.g., 2 kilograms), speed (e.g., 60 kilometers per hour), and distance (e.g., 500 meters). This non-directional nature simplifies their representation and interpretation.
Scalar quantities act as scaling factors or multipliers applied to vectors. For example, if we have a vector representing a displacement of 5 meters, multiplying it by a scalar value of 2 would result in a new vector representing a displacement of 10 meters.
Understanding the distinction between scalars and vectors is crucial in many fields, including physics, engineering, and mathematics. Scalars allow us to manipulate and scale vectors without altering their direction, enabling accurate calculations and analysis of physical phenomena.
Vector Operations in Mathematics
Since vectors include a magnitude and a direction, performing mathematical operations on them directly is not possible. So we need special math that only works with vector quantities. These are called vector operations. Let’s explore each operation.
Addition
Vectors can be added together component-wise. For two vectors 𝐮 = [𝑢₁, 𝑢₂, …, 𝑢ₙ] and 𝐯 = [𝑣₁, 𝑣₂, …, 𝑣ₙ], their sum is calculated as 𝐮 + 𝐯 = [𝑢₁ + 𝑣₁, 𝑢₂ + 𝑣₂, …, 𝑢ₙ + 𝑣ₙ].
Subtraction
Similarly, vector subtraction is performed component-wise. For 𝐮 and 𝐯, their difference is given by 𝐮 – 𝐯 = [𝑢₁ – 𝑣₁, 𝑢₂ – 𝑣₂, …, 𝑢ₙ – 𝑣ₙ].
Scalar Multiplication
A vector can be multiplied by a scalar, resulting in each component being multiplied by that scalar. If 𝐮 is a vector and 𝑘 is a scalar, then 𝑘𝐮 = [𝑘𝑢₁, 𝑘𝑢₂, …, 𝑘𝑢ₙ].
Dot Product
The dot product, also known as the inner product, is a binary operation that takes two vectors of the same length and produces a scalar value. If 𝐮 = [𝑢₁, 𝑢₂, …, 𝑢ₙ] and 𝐯 = [𝑣₁, 𝑣₂, …, 𝑣ₙ], then their dot product is given by 𝐮 ⋅ 𝐯 = 𝑢₁𝑣₁ + 𝑢₂𝑣₂ + … + 𝑢ₙ𝑣ₙ.
Cross Product
The cross product is a vector operation specifically defined for three-dimensional vectors. It produces a vector that is perpendicular to the two input vectors. If 𝐮 = [𝑢₁, 𝑢₂, 𝑢₃] and 𝐯 = [𝑣₁, 𝑣₂, 𝑣₃], then their cross product is given by 𝐮 × 𝐯 = [𝑢₂𝑣₃ – 𝑢₃𝑣₂, 𝑢₃𝑣₁ – 𝑢₁𝑣₃, 𝑢₁𝑣₂ – 𝑢₂𝑣₁].
Normalization
Normalizing a vector involves dividing each component of the vector by its magnitude (length). This results in a unit vector pointing in the same direction. If 𝐮 is a vector, then its normalized form is given by 𝑢̂ = 𝐮 / ||𝐮||, where ||𝐮|| represents the magnitude of 𝐮.
Scalar Projection
The scalar projection of a vector 𝐮 onto another vector 𝐯 gives the magnitude of the component of 𝐮 in the direction of 𝐯. It is calculated as 𝑢 ⋅ (𝐯̂) = ||𝐮|| cos(θ), where 𝐯̂ represents the normalized 𝐯, and θ is the angle between 𝐮 and 𝐯.
Vector Projection
The vector projection of 𝐮 onto 𝐯 gives a vector parallel to 𝐯 with a magnitude equal to the scalar projection. It is calculated as (𝑢 ⋅ 𝐯̂) 𝐯̂, where 𝐯̂ represents the normalized 𝐯.
Distance Metrics for Vectors
Distance metrics are critical for comparing vectors in machine learning. They measure the similarity or dissimilarity between data points, which is essential for tasks like clustering, classification, and recommendation systems. Here’s a detailed breakdown of the most commonly used distance metrics:
1. Euclidean Distance
Euclidean distance is the most common way to measure the distance between two points (vectors) in space. It is essentially the straight-line distance between them, calculated using the Pythagorean theorem.
Formula

Usage
In algorithms like k-means clustering, Euclidean distance is used to assign data points to clusters by calculating their proximity to the cluster’s centroid. It is also widely used in nearest neighbor algorithms, where the distance between a query point and other points determines classification or regression results.
2. Cosine Similarity
Cosine similarity measures the angle between two vectors rather than their straight-line distance. It is used to determine how similar two vectors are by focusing on their orientation rather than their magnitude. This makes it particularly useful for high-dimensional data, such as text, where the magnitude of the vectors may not be as important as the direction.
Formula

Where:
- u ⋅ v is the dot product of the vectors.
- ∣∣u∣∣ and ∣∣v∣∣ are the magnitudes (lengths) of u and v
Values:
- 1 means the vectors point in exactly the same direction (perfect similarity).
- 0 means they are orthogonal (no similarity).
- -1 means they point in opposite directions (complete dissimilarity).
Usage
In text analysis, cosine similarity is used to measure the similarity between document vectors or word embeddings, even if the vectors differ in magnitude. It is also used to find similar items (such as products or movies) based on user preferences or content.
3. Manhattan Distance (L1 Distance)
Also known as taxicab or city block distance, Manhattan distance measures the absolute differences between the components of two vectors. It calculates the distance a point would travel along the grid lines in a Cartesian plane, as if navigating through a city.
Formula

Usage
Manhattan distance is often used in image recognition tasks where pixel-by-pixel changes are more relevant than overall magnitude differences. Unlike Euclidean distance, Manhattan distance is less sensitive to outliers, making it a preferred choice when dealing with noisy or sparse data.
4. Minkowski Distance
Minkowski distance is a generalization of both Euclidean and Manhattan distances. It introduces a parameter ppp that allows you to adjust the sensitivity of the distance metric.
Formula
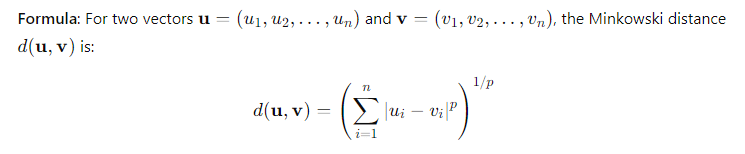
- When p=1, Minkowski distance becomes Manhattan distance.
- When p=2, it becomes Euclidean distance.
Usage
Minkowski distance is useful in cases where you want a flexible metric that can be fine-tuned to the specific characteristics of the data. Depending on the value of ppp, you can emphasize different types of distance relationships.
5. Hamming Distance
Hamming distance measures the number of positions at which the corresponding elements in two vectors are different. This metric is primarily used for categorical or binary data, such as comparing strings or binary vectors.
Formula

Usage
Hamming distance is commonly used in error detection and correction algorithms, where you want to identify how many bits in two binary sequences differ. It is also used for comparing strings and finding how many characters differ between two sequences, such as in DNA sequence analysis or text comparison.
Choosing the Right Distance Metric
Choosing the correct distance metric depends on the nature of your data and the task at hand:
- Euclidean Distance is effective for continuous data and works well in low-dimensional spaces.
- Cosine Similarity is ideal for high-dimensional data like text, where the magnitude of vectors is not as important as their direction.
- Manhattan Distance is robust to outliers and is preferred in grid-like structures such as image or geographical data.
- Hamming Distance is best for binary or categorical data, such as feature vectors with binary attributes or strings.
Understanding and selecting the appropriate distance metric is crucial to building accurate and efficient machine learning models, as it directly affects how your model perceives similarity or dissimilarity in the data.
Vectors in Machine Learning and Programming
In machine learning, data is often represented and organized using vectors. Each data point is typically represented as a vector, with each component of the vector representing a feature or attribute of the data. This vector representation allows machine learning algorithms to process and analyze the data effectively.
By organizing data into vectors, machine learning models can perform various operations on the data, such as clustering, classification, and regression. Vectors enable algorithms to leverage mathematical operations, such as calculating distances and similarities, to make predictions and learn patterns within the data.
In neural networks, vectors are often used as inputs, where each layer of the network transforms the input vector into a higher-dimensional representation. These transformations allow the model to learn complex patterns and relationships, leading to more accurate predictions.
Support Vector Machines
Support Vector Machines (SVMs) are a popular example of how vectors are used in machine learning algorithms. SVMs are used for classification tasks, where the goal is to divide data points into different categories or classes. SVMs find an optimal hyperplane that separates different classes in the feature space by maximizing the margin, or distance, between the classes.
In SVMs, data is represented as vectors, and the algorithm learns to identify a hyperplane that best separates the data points based on their feature values. SVMs use vectors and their relationships to find efficient decision boundaries, making them effective in handling complex classification problems.
The Vectorization of Data in AI
When working with AI and machine learning models, data must be represented in a format that algorithms can understand and process. Vectorization is the process of transforming data (such as images or text) into vectors so machines can perform mathematical computations.
In machine learning models, vectors are used to represent both the input data (features) and the output data (labels or predictions). Each data point is represented as a feature vector, where each component of the vector corresponds to a specific feature or attribute of the data.
For example, in image recognition, an image can be represented as a vector where each element represents the intensity of a specific pixel. In natural language processing, text documents can be vectorized using techniques like word embeddings, where each word is represented by a feature vector. Similarly, numerical data is often represented as vectors with each element corresponding to a specific numeric value.
By representing input and output data as vectors in machine learning, models can learn patterns, make predictions, and provide meaningful insights.
Vectors in Machine Learning Search Technology
Vector search provides several advantages in broad-based searches, especially when dealing with high-dimensional data.
- Vectors allow for efficient searches based on similarity. Similar items can be identified by measuring the distance or similarity between their vector representations. This enables quick retrieval of relevant and similar items, improving overall search performance.
- Vector search techniques can handle large-scale datasets and adapt to changes in the data distribution without significant performance degradation. This scalability makes vector search suitable for applications with growing databases and dynamic data.
- Vectors in machine learning can accommodate a variety of data types, including text, images, audio, and more. This enables multi-modal search capabilities, where users can search for information using different data modalities simultaneously.
Approximate Nearest Neighbor Algorithms
Vector search is often implemented using approximate nearest neighbor (ANN) algorithms. ANN algorithms are efficient at finding approximate nearest neighbors within large datasets. ANN algorithms strike a balance between accuracy and efficiency. They use techniques like indexing, hashing, or tree-based structures to retrieve similar vectors.
Vector Search and Semantic Search
Vector search and semantic search are complementary approaches that can be combined to enhance search capabilities. While vector search focuses on similarity-based retrieval using distance metrics, semantic search aims to understand the meaning and context of search queries and documents.
By combining these approaches, machine learning applications can leverage both the inherent similarities captured by vector search and the semantic understanding provided by semantic search. This synergy allows for more advanced search.
Enhancing Machine Learning with Vector Embeddings
Vector embeddings refer to the representation of objects or entities in a high-dimensional vector space. Each object is mapped to a dense vector, where similar objects are positioned closer to each other in the vector space. This captures semantic relationships and similarities between objects.
These n-dimensional space vectors are derived from machine learning algorithms that learn to encode features and capture patterns in complex data. Embeddings provide a way to transform raw data into a more compact and structured format.
The utility of embeddings lies in their ability to allow algorithms to leverage similarities and distances between vectors to make predictions, perform recommendations, or analyze text and image data.
Deep-Learning-Powered Embeddings
Deep learning has revolutionized the field of embeddings, enabling the creation of more sophisticated and powerful representations. Deep learning models, such as neural networks, have the ability to learn and extract intricate patterns and representations from data.
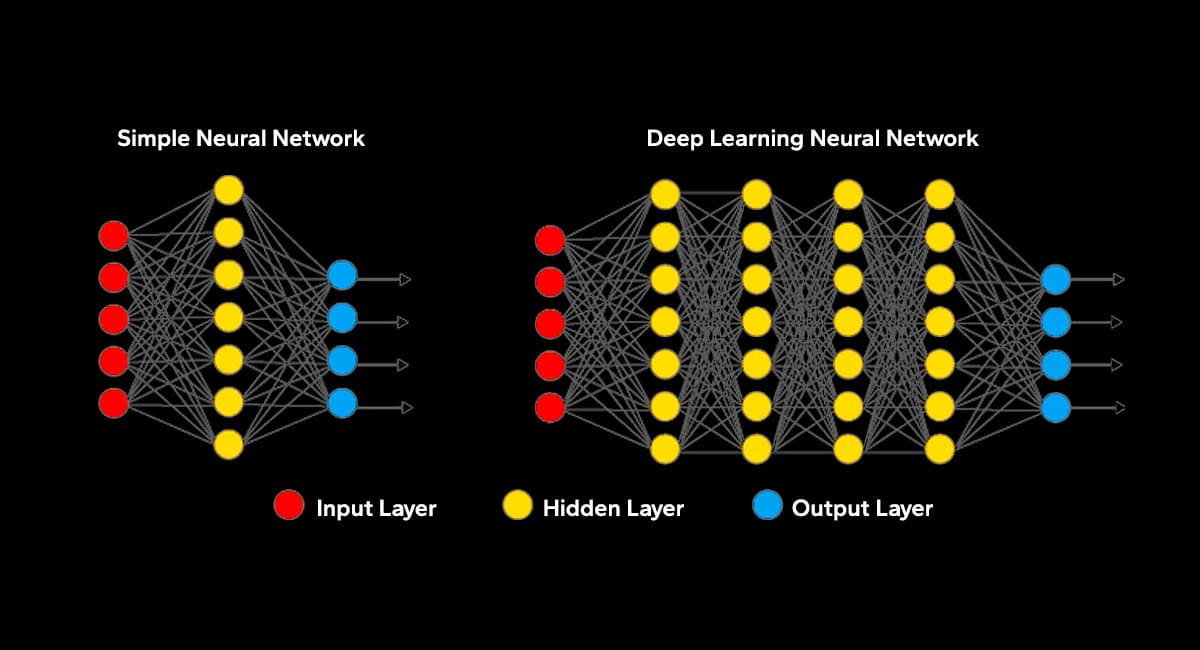
Deep-learning-powered embeddings have had a significant impact on various machine learning tasks. For example:
- Embeddings are instrumental in natural language processing tasks like machine translation, sentiment analysis, and named entity recognition. Algorithms can learn to embed words, phrases, or whole documents as vectors.
- Image embeddings have significantly advanced image recognition tasks, enabling accurate object detection, image captioning, and visual search.
- Embeddings have improved recommender systems that provide personalized recommendations. Algorithms can measure similarity and make relevant recommendations based on past user behavior and preferences.
The introduction of deep-learning-powered embeddings has enhanced the performance and capabilities of machine learning algorithms across domains. These embeddings enable models to capture complex patterns, generate meaningful representations, and achieve state-of-the-art results in various tasks.
Vector Embeddings in NLP
Vector Embeddings in NLP In natural language processing, models like Word2Vec and GloVe transform words into vectors. These word embeddings allow words with similar meanings to be positioned close to each other in vector space. This helps algorithms perform tasks like sentiment analysis or topic modeling more effectively by comparing the proximity of word vectors.
Neural Hashing: Streamlining Vector Processing
High-dimensional data often leads to lengthy vectors. Longer vectors means higher storage requirements and lower computational efficiency of machine learning algorithms, especially when dealing with large datasets.
Furthermore, when faced with similar or redundant information within vectors, algorithms may encounter overfitting issues, which can hinder generalization and accuracy.
Neural hashing offers a solution here. It aims to project high-dimensional vectors into compact binary codes, referred to as hashes. This compression allows for more efficient storage and faster computation, as binary codes require significantly less memory compared to their original high-dimensional counterparts.
Neural hashing involves training deep neural networks to learn the mappings that transform long vectors into compact hashes. These mappings capture and preserve important information present in the original vectors. Then various machine learning tasks can be performed directly on the compressed binary codes.
The benefits of neural hashing are twofold. First, it reduces the memory and storage requirements for vectors, allowing for faster and more efficient use of resources. Second, by mapping similar vectors into close binary codes, it enables efficient similarity searches, retrieval, and clustering in large datasets.
Neural hashing has various applications, including image retrieval, recommendation systems, and information retrieval. It’s an efficient and effective solution to streamline vector processing, compress lengthy vectors, and overcome computational challenges in machine learning tasks.
Challenges and Limitations of Vectors
While vectors are powerful tools in machine learning and AI, they come with certain challenges and limitations that you should be aware of.
Curse of Dimensionality: As the number of dimensions in a vector increases, the distance between points becomes less meaningful. In high-dimensional spaces, data points may appear equidistant from one another, making it harder for algorithms to differentiate between them. This can reduce the effectiveness of clustering and classification techniques.
Scalability: When working with massive datasets, storing and processing high-dimensional vectors becomes computationally expensive. Handling such data may require significant memory and computational power, which can slow down machine learning algorithms and increase resource costs.
Interpretability: High-dimensional vectors can be difficult to interpret, especially when dealing with abstract data like word embeddings in NLP or feature vectors in image recognition. Understanding the significance of individual components of a vector is not always straightforward.
Loss of Information in Vectorization: In certain cases, converting complex data (such as images, text, or audio) into vectors may lead to a loss of information. Simplifying rich, multi-faceted data into a mathematical format can result in the omission of context or nuances.
Vector Representation Choice: Choosing the right vector representation is critical for the success of machine learning models. Poor representation can lead to incorrect or biased results, as the vector may fail to capture the relevant features of the data. This challenge is especially prominent in fields like natural language processing, where subtle differences in word meaning may not be fully captured by a vector.
Addressing these challenges often requires additional techniques, such as dimensionality reduction, more efficient storage methods, or careful tuning of machine learning models to ensure accurate results without excessive computational overhead.
The Value and Versatility of Vectors in Machine Learning
By now you should understand the value and versatility of vectors in machine learning. Vectors provide a powerful framework for representing and manipulating data. They allow for efficient organization, processing, and comparison of data in high-dimensional spaces. They are indispensable in machine learning, accommodating different data types and supporting efficient operations on large-scale datasets.