Like many businesses, you’re probably inundated with an unprecedented volume of structured and unstructured data. The challenge now is not just about storing your data, but managing, classifying, and transforming it into fuel that your business’ engine can use.
Generative AI and other AI applications have made this more important than ever for organizations and business leaders of any type.
In this article, we will explore the key steps to prepare your data for generative AI applications.
What is Generative AI for Business?
Generative AI isn’t just a buzzword—it’s a real game-changer for businesses looking to innovate. Countless organizations are embedding it into your business operations.
At its core, generative AI refers to algorithms that can create new content, whether it’s text, images, or even entire strategies. It’s like having an intelligent assistant that not only answers questions but also crafts original responses and insights.
For businesses, this means you can automate content creation, streamline customer interactions, and even design new products.
Business Applications of Generative AI
Generative AI models have countless applications across different industries, and many companies are already exploring its potential. Here are just a few ways it’s being used:
- Customer Support: Imagine a chatbot that doesn’t just give generic answers but understands the specific needs of your customers. Generative AI tools use your company’s knowledge base to provide responses that are far more personal and meaningful.
- Content Generation: Whether you need social media posts, blog articles, or email drafts, generative AI can create content that matches your brand voice. It’s all about cutting down the time it takes to create engaging content while maintaining quality.
- Product Design: Companies are using generative AI tools to design new products. Think of it as a digital collaborator, offering innovative designs by analyzing patterns and customer feedback. It speeds up the design process and often results in creative solutions that might not be obvious to a human.
Use Cases Across Industries
Generative AI isn’t just about the tech—it’s about how it can transform the way you work. It gives you the ability to create smarter customer experiences, make better decisions, and unlock the true potential of your data. To that end, it’s being used across a range of industries to solve real problems.
In healthcare, generative AI helps in creating personalized treatment plans. Doctors can use generative AI to create accurate insights based on patient history to make better, data-driven decisions.
Banks are using generative AI to draft customer reports, create market analysis summaries, uncover valuable insights, and even generate personalized financial advice. It saves time for analysts and makes services more accessible to customers.
Retailers are turning to generative AI to enhance customer experiences, from generating personalized product recommendations to creating virtual shopping assistants that can chat with customers about the latest styles.
In manufacturing, AI is being used to optimize supply chains. By predicting demand and creating production schedules, generative AI helps reduce costs and streamline operations.
“Garbage In, Garbage Out”
You’ve probably heard the phrase “Garbage In, Garbage Out” before. It’s a simple idea but a critical one when it comes to AI.
If you feed an AI system bad data, you’re going to get bad results. The quality of the output is only as good as the quality of the input. For businesses thinking about leveraging generative AI, this means that having messy, incomplete, or irrelevant data can lead to AI models that don’t deliver value.
Importance of Data Quality for AI Outcomes
Data quality is the foundation for any successful AI project. If your data is inconsistent or filled with errors, your AI isn’t going to magically fix that—it’s just going to amplify the problem.
Imagine trying to use an AI chatbot for customer service, but the data it draws from is outdated or full of gaps. Instead of providing a helpful answer, the bot might give irrelevant advice or even frustrate your customers with incorrect information. That’s not just bad AI; that’s bad for your brand.
High-quality data, on the other hand, gives AI the context it needs to understand what’s going on and make informed predictions. When your data is clean, accurate, and up-to-date, the AI can perform at its best—producing insights that actually help you make smarter decisions, enhance customer experiences, and streamline operations.
Examples of Poor Data Quality Impacting AI Performance
What is the impact of poor-quality data? Let’s look at some examples.
Customer Service Mishaps: Imagine a customer is contacting support about an issue they’ve had before, but your AI chatbot can’t access complete interaction histories. Without that full picture, the bot might recommend a solution the customer has already tried, leading to frustration and an escalated call to human support.
Marketing Campaign Blunders: If your customer data has errors, like incorrect contact information or outdated preferences, an AI used for personalized marketing might send the wrong content to the wrong people. Suddenly, your carefully crafted email campaigns are irrelevant at best—or annoying to your audience at worst.
Faulty Predictions in Finance: In financial services, AI models depend on clean data to predict market trends or detect fraud. If the data fed to these models is messy or incomplete, you might end up making poor investment decisions or failing to spot suspicious activities.
How to Prepare Your Data for Generative AI
Getting ready for generative AI isn’t just about picking the right technology—it’s about making sure your data is up to the task. Without the right preparation, even the most powerful AI won’t deliver the results you’re hoping for.
This section will guide you through the steps needed to make sure your data is ready, from defining your goals to establishing a solid foundation for quality data. Let’s dive into how you can get your data in shape for generative AI.
1. Define Your Project Goals
Before you start gathering data or picking an AI model, you need a clear understanding of what you want to achieve. Generative AI can do a lot of amazing things, but if you’re not aligned on what problem you’re solving, you’ll end up going in circles.
Start by asking yourself: What’s the core business problem you’re trying to solve with AI? Whether it’s improving customer experience, optimizing processes, or generating new content, your objectives should guide every decision you make.
Aligning your goals with what AI can realistically deliver ensures that you’re focusing on the right data and setting achievable expectations. But it’s not enough to say you want “better results”—you need to define what success looks like in terms of metrics.
Metrics could be as simple as reducing customer service response times or increasing user engagement by a specific percentage. Clear metrics help you measure your progress and understand whether the AI project is delivering value.
2. Establish a Data Lakehouse
Your data infrastructure is key to generative AI success. A data lakehouse is a modern approach that brings together the best of data lakes and data warehouses, making it easier to store both structured and unstructured data all in one place.
This kind of unified storage is especially useful for generative AI, which typically needs a wide variety of data sources to perform well.
Structured vs. Unstructured Data
Understanding the types of data you have is crucial. Structured data includes everything neatly organized in tables—like customer records or transactional data. Unstructured data, on the other hand, could be anything from emails to social media posts.
Generative AI thrives on both types, so having a system that can handle them efficiently is a must. Explore these two types of data here: Structured vs. Unstructured Data.
Natural Language Processing (NLP)
Unstructured data can be messy, but it’s incredibly valuable. That’s where natural language processing comes in. These tools are language models that help your AI make sense of human language, extracting insights and context from unstructured sources.
By leveraging NLP, you can transform raw text into useful data, giving your generative AI a richer understanding of your business.
3. Clean and Organize Your Data
Once you have your data stored in a lakehouse, the next step is to clean it up. Like we mentioned earlier, generative AI is only as good as the data it’s fed, so data quality is non-negotiable. Messy data—full of errors, inconsistencies, or duplicates—can lead to unreliable AI outputs.
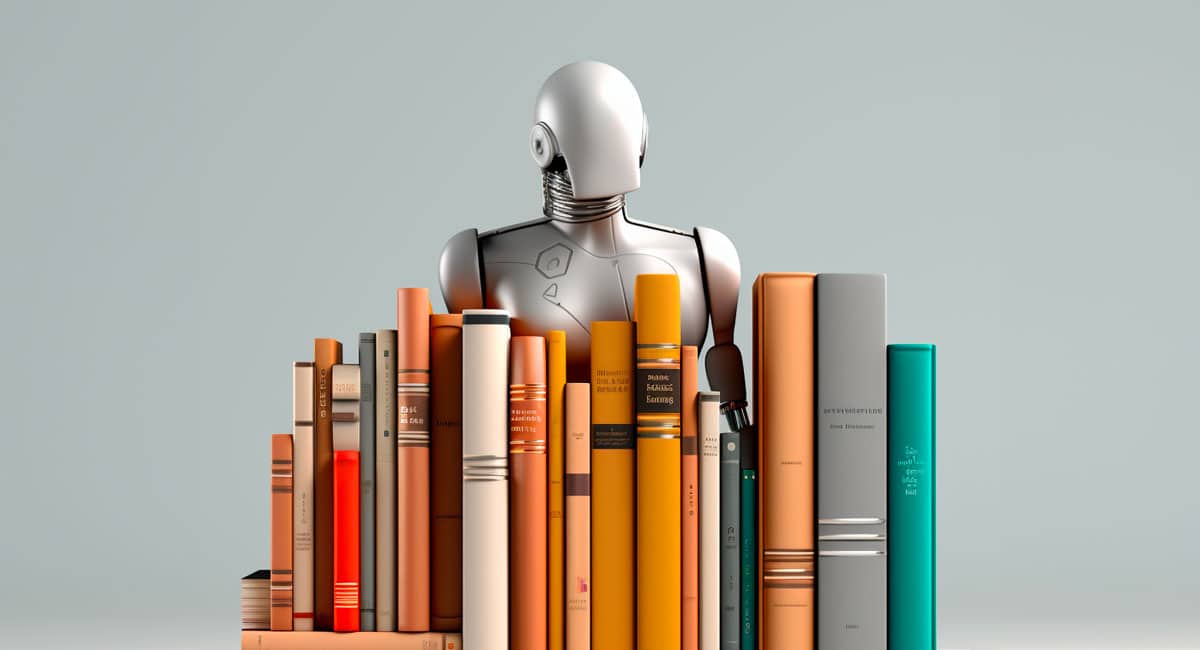
Data consistency is about making sure that information is up-to-date and standardized across your systems. If one part of your business is using outdated customer records while another has newer data, your AI is going to get confused. Ensuring that data is consistent helps your AI provide accurate and useful results.
Sounds like a lot of work? You’re right. Manually cleaning data is not only time-consuming but also error-prone. Automation tools like Shelf’s unstructured data management platform can help take this load off your data team. This tool can spot inconsistencies, flag duplicates, and ensure your data meets the quality standards you set—giving you confidence that what goes into your AI is reliable.
4. Boost Your Data Collection
Once you’ve got your existing data cleaned and organized, it’s time to think about expanding your data collection. Generative AI works best when it has a wide range of high-quality data to learn from—so improving your data coverage is key.
Improving data coverage means looking at where your data is coming from and whether it’s enough to support the kind of insights you need. Are you getting data from all the touchpoints you should be? Expanding your data collection efforts can help you capture a fuller picture of your customers, your operations, and your business environment.
Collecting more data is also about breaking down silos. If your data is stuck in silos—separated by department, system, or region—it’s hard for your AI to get the full story.
AI models work best when they have a unified view of all available data, allowing them to see connections that humans might miss. Unified access also means less duplication, fewer inconsistencies, and ultimately, better AI outcomes.
Overcoming data silos involves making sure all your data sources are connected so that your AI has access to everything it needs. The more integrated your data, the better your AI can provide meaningful, actionable insights.
5. Integrate Retrieval-Augmented Generation (RAG)
When you integrate generative AI with a Retrieval-Augmented Generation (RAG) system, it gets even smarter.
RAG is a technique that enhances your AI by feeding it real-time data from reliable internal sources, which means your AI isn’t just generating responses based on what it learned during training—it’s also pulling in up-to-date, business-specific information.
The benefits of RAG for data readiness are substantial. It allows your AI to generate more accurate, relevant answers because it’s not limited to static knowledge.
For example, instead of giving a generic troubleshooting guide to a customer, RAG can make your chatbot aware of specific issues—like a regional outage or a recent support ticket—so it can give tailored advice. This makes customer interactions much more effective and reduces frustration for users.
6. Safeguard Sensitive Data
AI can’t thrive if it puts sensitive information at risk, especially with increasing regulations around data privacy issues. Safeguarding your data means being mindful of where it’s stored, how it’s used, and who has access to it.
Data privacy and compliance considerations should be at the forefront of your AI projects. You need to ensure your AI systems are compliant with industry regulations, like GDPR or CCPA, depending on your region and industry. This includes making sure that personally identifiable information (PII) is protected at every stage.
To protect sensitive data, you’ll want to use techniques like data masking and anonymization. Data masking involves hiding certain parts of the data so that sensitive information isn’t exposed, while anonymization removes identifiable attributes to ensure individuals can’t be traced back from the data.
These techniques help you strike the balance between data usability and privacy so your AI has the data it needs without compromising security.
7. Select and Train a Model
With your data in order, it’s time to focus on choosing and training the right AI model for your project. Not all models are created equal, and the best one for you depends on what you want to achieve.
Choosing the right AI model means considering factors like the complexity of your data, the problem you’re solving, and how much computing power you have available. If you need something quick and reliable, you might go with a pre-trained model. These models are already trained on massive datasets and can be adapted to a range of tasks with minimal effort. They’re great if you want to get started fast without needing a lot of custom training.
However, if your use case is more specific—say, you need an AI that understands niche industry jargon or unique customer behaviors—you might consider fine-tuning a model. Fine-tuning involves taking an existing pre-trained model and training it further using your own data to make it more specialized. It takes more time, but the result is an AI model that’s far better suited to your unique needs.
8. Monitor and Maintain Data Quality
Getting your data ready for generative AI is a big step, but the work doesn’t stop there. Data quality isn’t something you can just check off your list—it requires ongoing attention. Your data landscape is constantly changing, and if you want your AI to keep producing reliable results, you need to make sure the quality stays high.
Establishing ongoing quality checks is essential. These checks should be built into your data workflows to catch issues like missing values, inaccuracies, or outdated information before they impact your AI. Regular quality audits help ensure that your data remains consistent and useful.
You can also use AI agents to help manage data quality. Shelf’s content intelligence agents can automate a lot of the grunt work involved in quality maintenance, like flagging anomalies or standardizing data formats. This keeps your data clean without overwhelming your team with manual tasks.
9. Test and Validate AI Outputs
The final step before you roll out your AI is to test and validate its outputs. Even the best-trained AI models can produce unexpected or incorrect results, so it’s critical to set up feedback loops to catch issues early.
Feedback loops allow you to gather input on how well the AI is performing and make adjustments as needed. This continuous learning helps you improve accuracy and ensure that the model is providing real value.
One practical approach to validating your AI is to use smoke tests. Smoke tests are quick, preliminary tests to see if your model’s basic functions are working as expected. They help identify glaring issues without the need for a full-scale evaluation.
Building a Healthy Data Foundation
Preparing your data for generative AI might seem like a big undertaking, but it’s a crucial part of making sure your AI delivers real, impactful results. Every step of the process we outlined above helps you build a solid foundation that your AI can thrive on.
Remember, the quality of your AI outputs is directly tied to the quality of the data you put in, so taking the time to clean, organize, and enhance your data isn’t just an extra step.
As you go through the process, keep in mind that this isn’t a “set it and forget it” journey. Your data will continue to grow and evolve, and maintaining data quality, breaking down silos, and ensuring privacy will be ongoing responsibilities.
By following these steps and with some careful planning, you’re not just preparing your data for today’s generative AI—you’re setting up a strong data foundation that will allow you to keep innovating, adapting, and staying competitive.
Shelf’s unstructured data management platform offers several generative AI-readiness tools that keep your data quality high so it creates maximum impact when used with AI models.