The rapid advancement of artificial intelligence (AI) provides unprecedented opportunity for innovation and greater productivity, but an important question remains: how do you control AI? Businesses eager to harness the power of AI know the potential of the technology, but providing AI complete access to proprietary knowledge or private customer information requires a guarantee of control. We’ve talked about the importance of control (as well as transparency and trust) and in this blog we’ll dig into how to control AI. We’ll explore how businesses can leverage AI’s potential while mitigating unforeseen risks. It all begins with knowledge management.

Unleashing the Potential of AI
Much of the potential of AI comes from large language models (LLMs) and more specifically generative artificial intelligence (GenAI). This technology has the ability to learn, adapt, and offer innovative solutions to complex problems. These abilities are what make AI so promising, but also the source of concerns over risk. Risk can be specific to security or privacy, but generally it is the risk the technology will do something unpredictable. Generally, businesses want to know exactly how new technology will change or influence their current operations.
What is Knowledge Management?
Knowledge management (KM) is the process of capturing, organizing, storing, and sharing knowledge within an organization to enhance its overall effectiveness and decision-making capabilities. It involves the systematic management of information on an ongoing basis. Check out our guide on knowledge management.
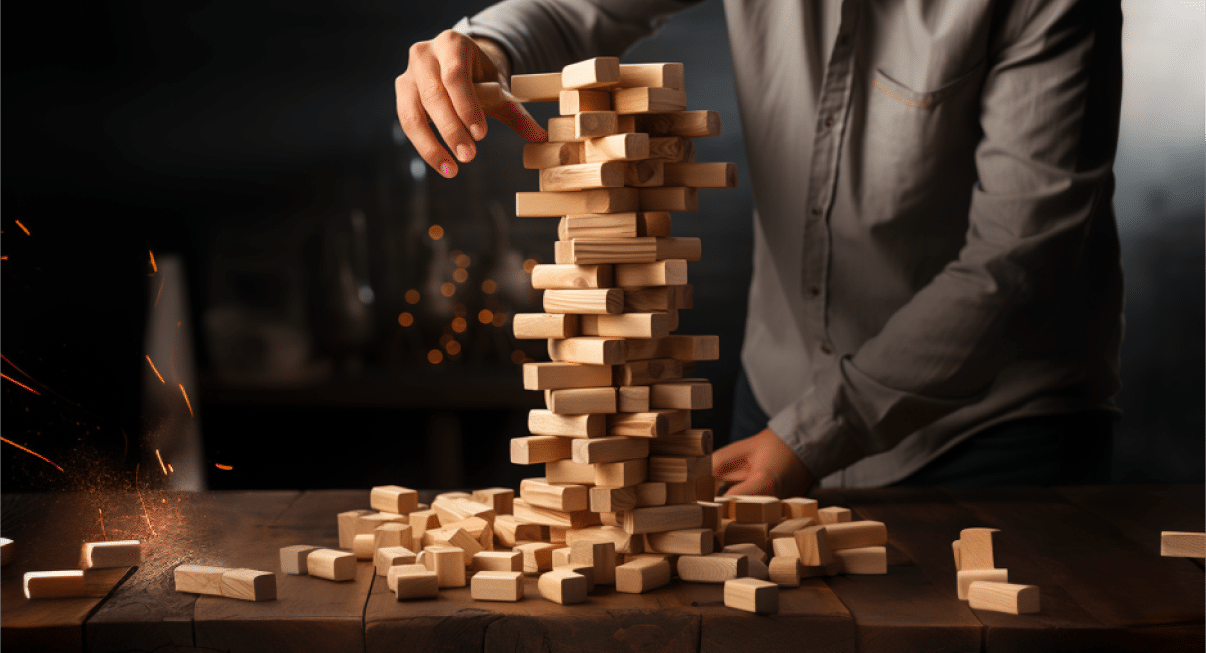
Controlling the Risks
Risk relating to AI is the same as any other type of risk: it can be mitigated with proactive measures. We’ve bucketed these forms of risk mitigation into broad terms that can be used as a reference for any industry.
Minimize complexity
Many AI technologies present as a tool that can do virtually anything. This goes along with many AI evangelists who talk about wide-ranging AI implementations to fundamentally change a business (if not society itself). While this sounds very exciting, it is an easy starting point for mitigating risk. Depending on your business, you don’t necessarily need an AI that oversees every department, office, and function while communicating within all of those entities. The level of complexity of your AI should match the specific operations it is intended to support.
For example, if you’re utilizing AI to support knowledge management to make it easier to retrieve information within your organization, you don’t need to connect that specific AI product to other tasks augmented with AI.
Imagine a healthcare company that uses AI to assist in accessing patient insurance information. This healthcare company may also use AI to assist doctors and provide diagnostic tools to identify potential illnesses or recommend treatments. These are two distinct use cases of AI that do not need to communicate with one another. Limiting the complexity of the AI’s knowledge can eliminate the possibility of protected information slipping into the wrong place.
You can envision the concept of minimized complexity as two different spectrums. How much access does the AI have to your knowledge and how much can it generate based on that knowledge? An AI used for insurance information may have a lot of access but no need to generate content. Whereas a diagnostic AI may generate content but not need access to other knowledge. On their own these are two distinct risks that can be easily mitigated, but combining them would create far more unnecessary exposure.
Cultivating Responsible Control
For the foreseeable future, AI will operate with the oversight of a human in the loop. This is the term used for having a member of your team dedicated to ensuring the outputs from AI are accurate and valuable. This may sound like a position meant for a technologist — or someone well-versed in AI engineering, but that isn’t necessarily the case.
Different departments have different concerns when it comes to mitigating risk. Perhaps more importantly, the concept of responsible control needs to be integrated into the culture of the organization. Stakeholders should be aware how AI may affect their role or department and share that information internally. How AI impacts the goals you have for your team within an organization isn’t something an AI engineer would know unless there is cross-disciplinary input on how to cultivate this control.
This process may take a number of test scenarios to see how it plays out. This is new technology so organizations will need to explore how different controls result in different outcomes. Teams should be mindful of unexpected behavior. Ideally, your AI technology provides the opportunity to audit how an undesirable outcome was produced in the first place.
Building a Machine Conscience
This may sound like you’ve stepped through the looking glass, but some of the problems of AI can be resolved with more AI. Imagine a parallel system of two AI entities where one produces content and the other checks it within criteria such as laws, corporate policy, cultural norms, or other rule sets. This secondary AI can act as a “machine conscience.”
The machine conscience would prevent your AI from breaking the law, violating company standards, or accidentally acting on bias within the workplace. A singular conscience could review what an AI produces, but it may also be worth splitting up this process so there is one dedicated conscience for each consideration. The purpose of dividing this process across multiple AIs is to allow the initial work to be produced without restrictions then process it to your organization’s requirements.
For larger organizations, this initiative may require an in-house team of AI designers. This would include attracting high-quality AI specialists who can shape your own organization’s AI — based off of other tools available today.
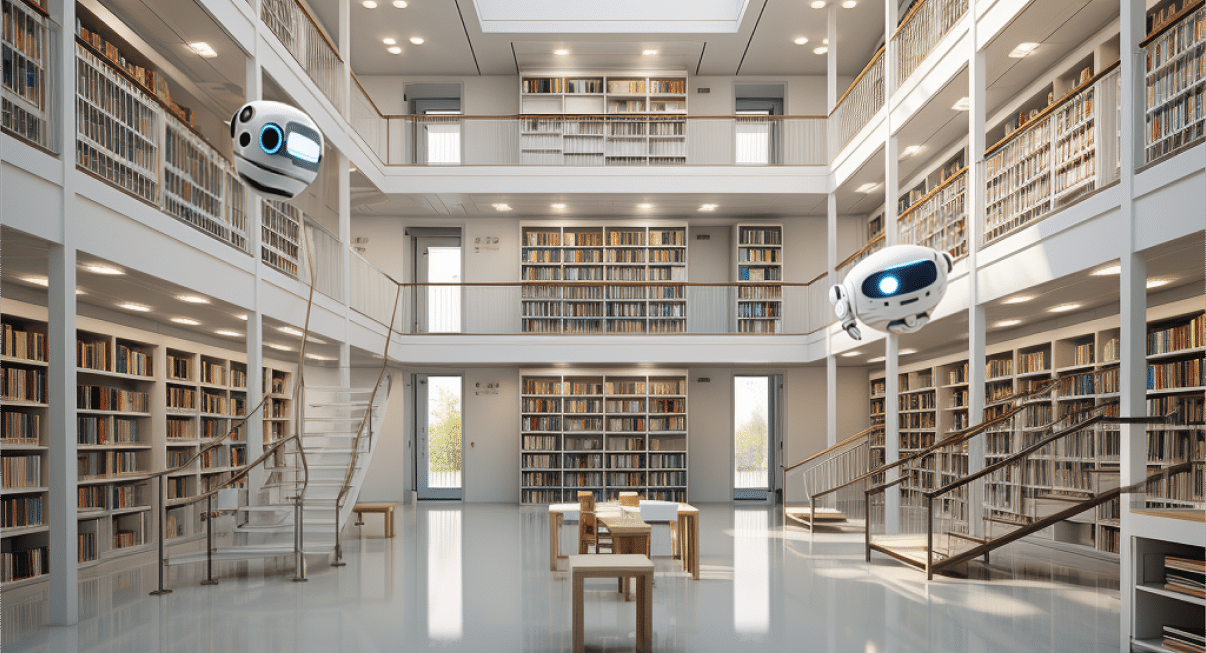
The Role of Knowledge Management
All of these risk mitigation efforts can be addressed with knowledge management. Afterall, AI produces its answers based on what knowledge it can access. Maintaining your knowledge base and ensuring it is accurate, reliable, and aligned with your organization’s desired outcomes will contribute to the success of mitigating risk. The answer to “how do you control AI” begins with a robust knowledge management strategy.
How Do You Control AI?
Harnessing the potential of AI will make the difference for competing organizations in the future. The opportunities made possible by this technology come with a number of new risks, but by following the steps outlined in this blog — and giving knowledge management the priority it deserves — business leaders can navigate the potential and risks of this new technology. Navigating AI will be integral to deploying the digital transformation required to achieve business objectives guided by the productivity gains made possible by this technology.
It is important to remember AI’s accuracy and reliability is shaped by the data it can access. By providing accurate and reliable information, organizations can minimize unintended consequences and mitigate the risks that come with new technology.