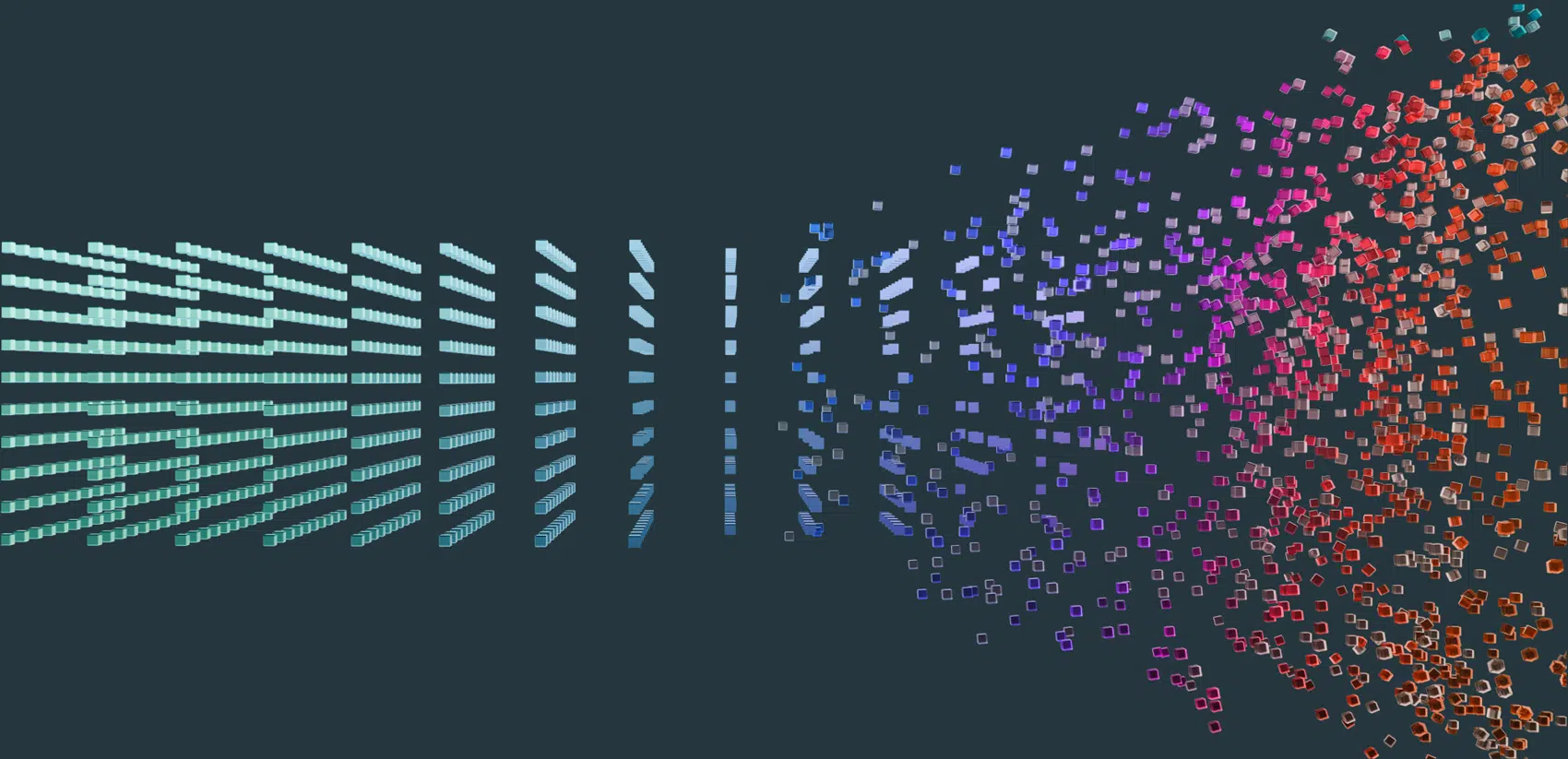
Overview of unstructured data entropy
Data entropy is the gradual decline in the quality of an organization’s unstructured data and content over time. It happens inside every organization, and results in sections of documents and files (unstructured data) going out-of-date, becoming inaccurate, and becoming noncompliant.
The major cause of data entropy is simple: the number of files that companies have to manage is growing exponentially. According to Infosecurity Magazine, the average employee has access to 10 million files and this number grows every year.
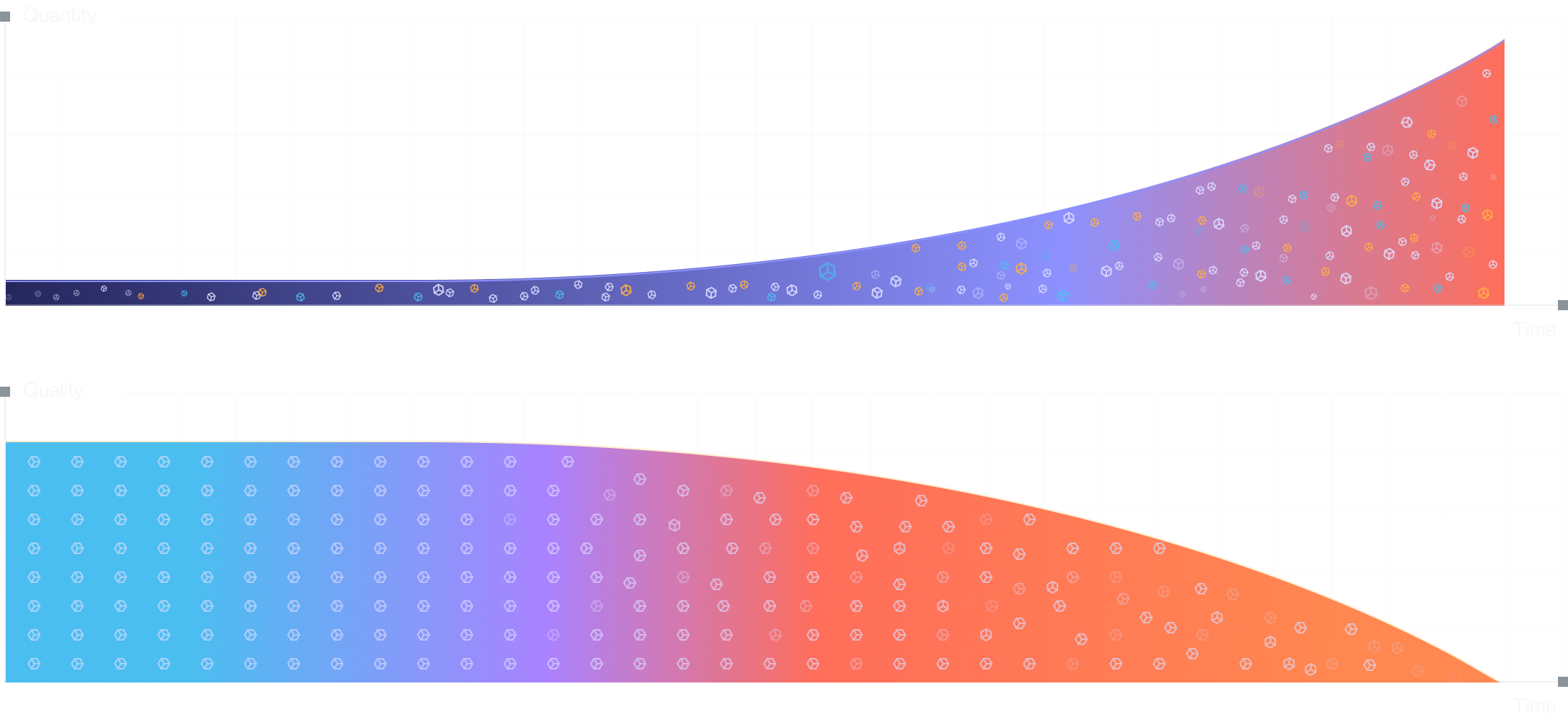
Yet most companies have a limited number of people responsible for managing and updating this mountain of unstructured data. Most organizations have less than 10 full-time people dedicated to content management and this number isn’t growing. This means that a small number of people are responsible for keeping track of, updating, archiving, and maintaining millions of documents. This is an impossible task for thousands of people, let alone less than a dozen.
Furthermore, we live in a world of accelerating change with a dynamic, constantly changing market. This means that businesses, and all their supporting documents, also need to constantly change. Unfortunately, content teams are unable to keep up, and as a result sections of documents and media files are continuously going out of date and becoming inaccurate.
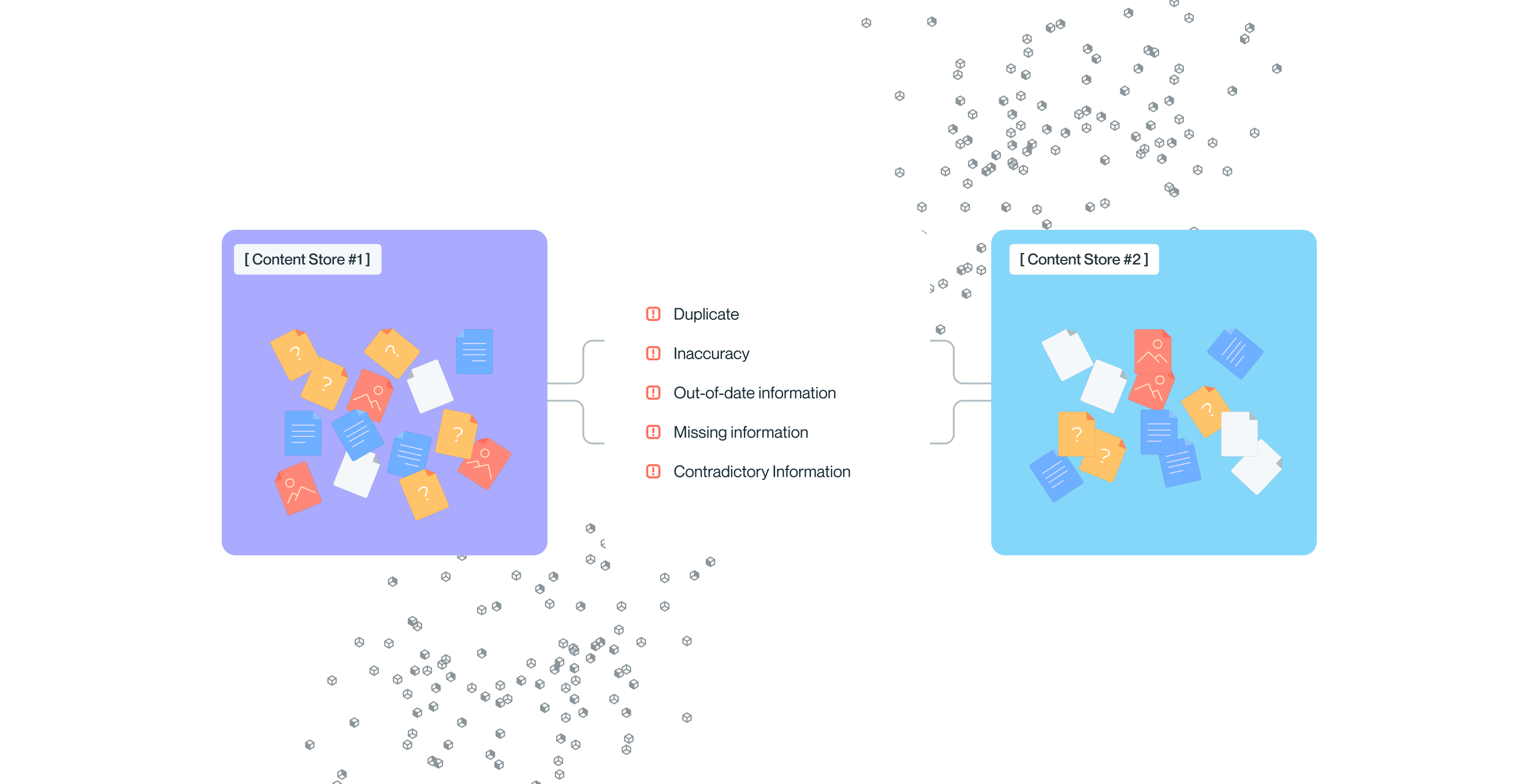
The unstructured data entropy statistics
The statistics around the quality of organizational knowledge are shocking. Overall, 82% of files have at least one major risk and both people and bots are consuming these files every day, using them to answer both internal and customer questions.
Impacts of data entropy
According to a poll of IT leaders by Gartner, poor data quality is the #1 obstacle companies face in their GenAI initiatives. Unstructured data makes up over 90% of organizational data. As the saying goes, “Garbage In, Garbage Out”, and without a strategy in place to fix the root cause of this issue, companies are going to continue to struggle implementing AI at scale.
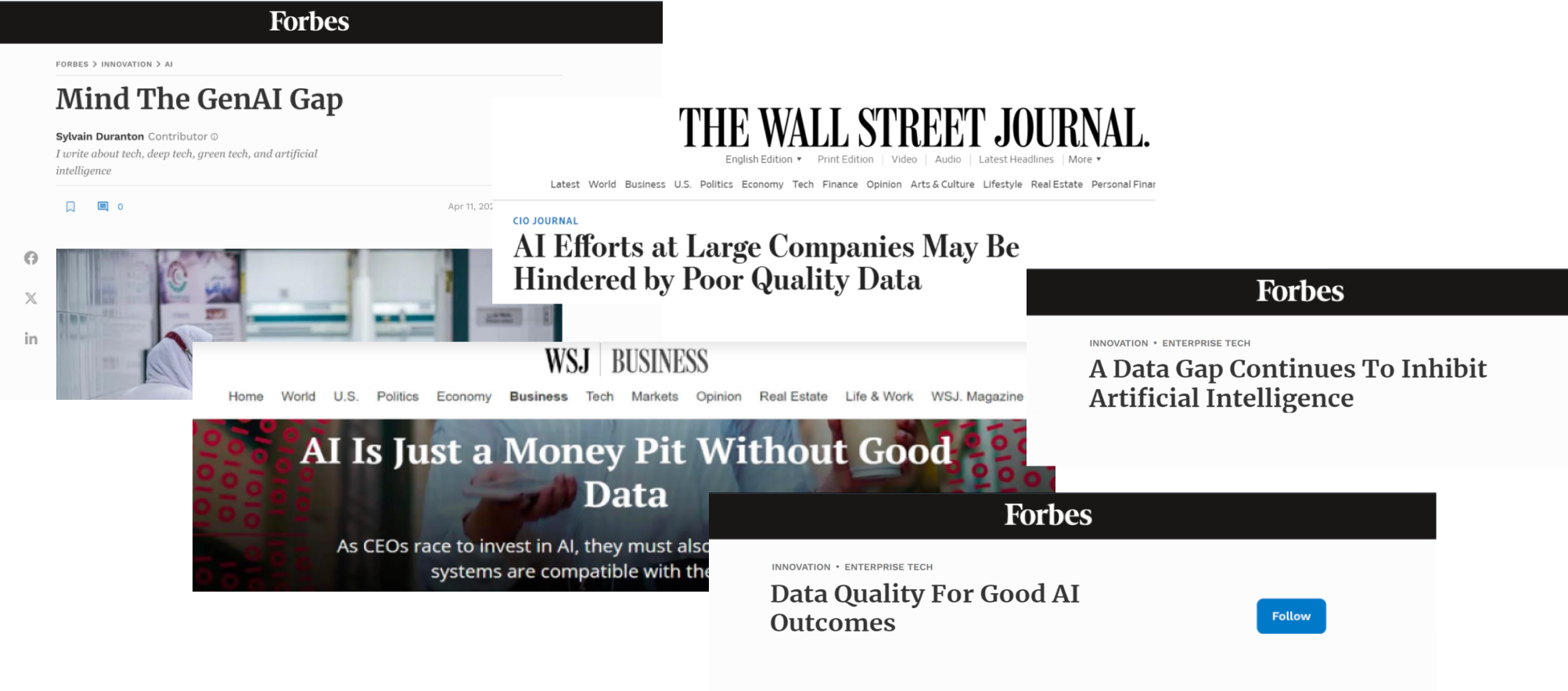
Poor quality unstructured data impacts AI initiatives and GenAI in a number of ways:
Factual inaccuracies and/or hallucinations
Causing customers or employees to take the wrong actions
Risk of toxic/biased outputs
Privacy/compliance violations
Customer dissatisfaction
Brand reputation risks
Unfortunately, most AI strategies ignore unstructured data quality issues and instead rely on over-engineering, manual tasks that don’t scale, and overspending with little ROI to show for it. Some of things that companies are doing that won’t work in the long run include:
- Out-of-the-box Retrieval Augmented Generation – Limited to text chunking with minimal customization options and no content enrichment or quality controls.
- Optimizing LLM settings – requires significant technical work and does not impact content quality.
- Adding processing layers on top of LLMs, a highly manual and non-scalable solution.
- Fine-tuning on their own data – a costly solution requiring a substantial technical and manual effort with unpredictable results.
- Building predefined Q&A lists – In addition to requiring a lot of manual work, this is a highly rigid solution with limited knowledge coverage.
- Raw content chunking and vectorization (embeddings) – Fails to preserve contextual information and does not process tables or images.
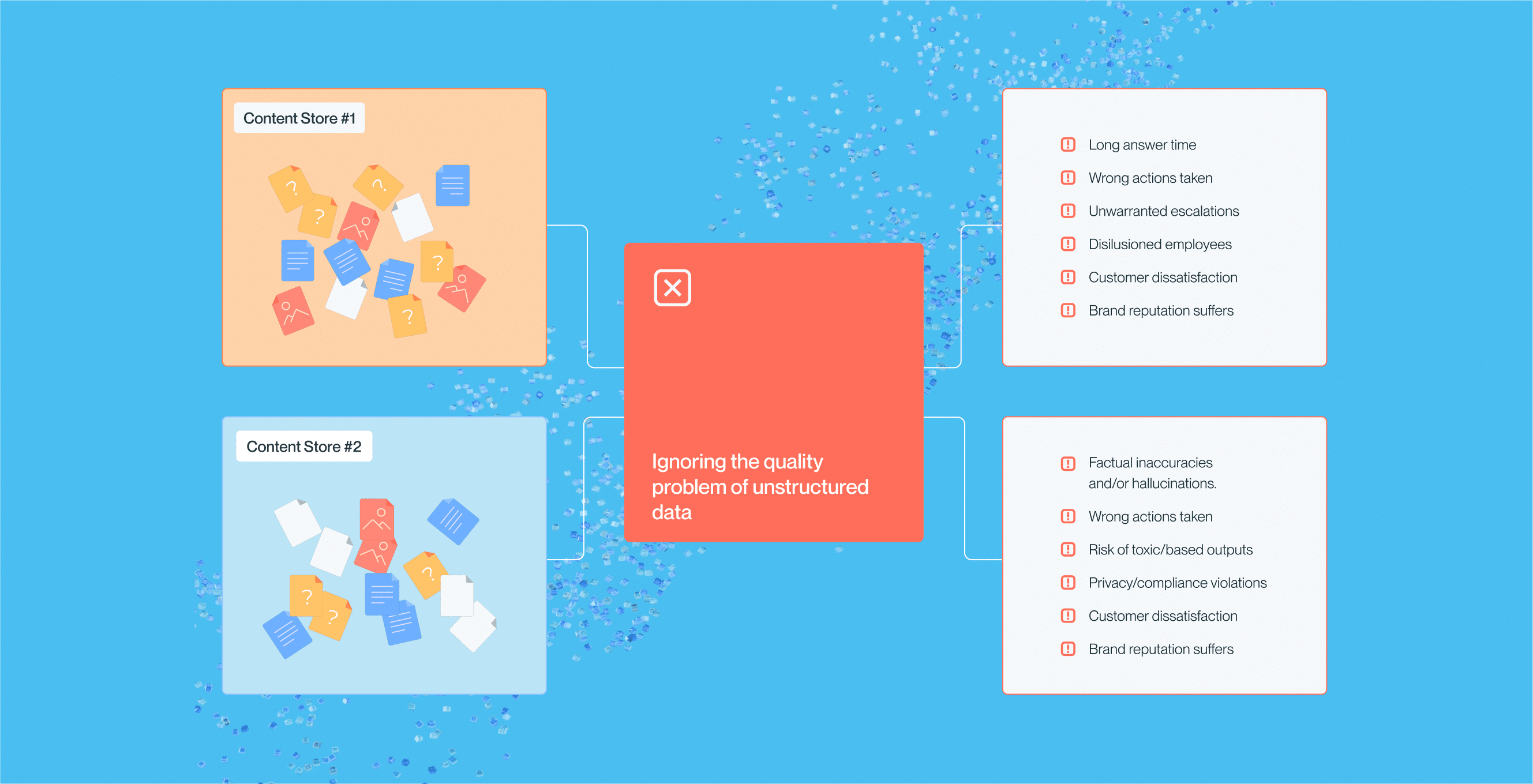
And AI is not the only casualty of low-quality unstructured data. People consume just as much inaccurate and out-date content as machines. This issue has existed since the information age began. It’s just been harder to measure and thus, harder to prioritize.
The practice of Knowledge Management was created to keep content accurate, up-to-date, and trusted. And for the most part, it hasn’t fulfilled its promise because of the ever-growing black box of content quality issues.
Unstructured data issues that impact people include:
- Time lost trying to track down the correct version.
- Wrong information being used, leading to the wrong actions being taken.
- The unnecessary escalation of issues that could have been solved by finding answers earlier.
- Inappropriate access to sensitive and noncompliant information.
- Customer dissatisfaction and brand reputation risks.
Unstructured data is the fuel of the AI Age, and for the vast majority of companies, this fuel is unrefined and dirty. Without a process of refining and cleaning this data it is unusable at best and dangerous at worst, generating inaccuracies that can cause harm to the business rather than enabling it to thrive.
Solving company data entropy
It doesn’t have to be this way. By combining the best practices from data management and knowledge management, a more analytical, automated, and intelligent approach can be applied to data entropy issues. A content quality control layer is needed to guard against inaccurate, out-of-date, and noncompliant content being fed into AI or human use cases.
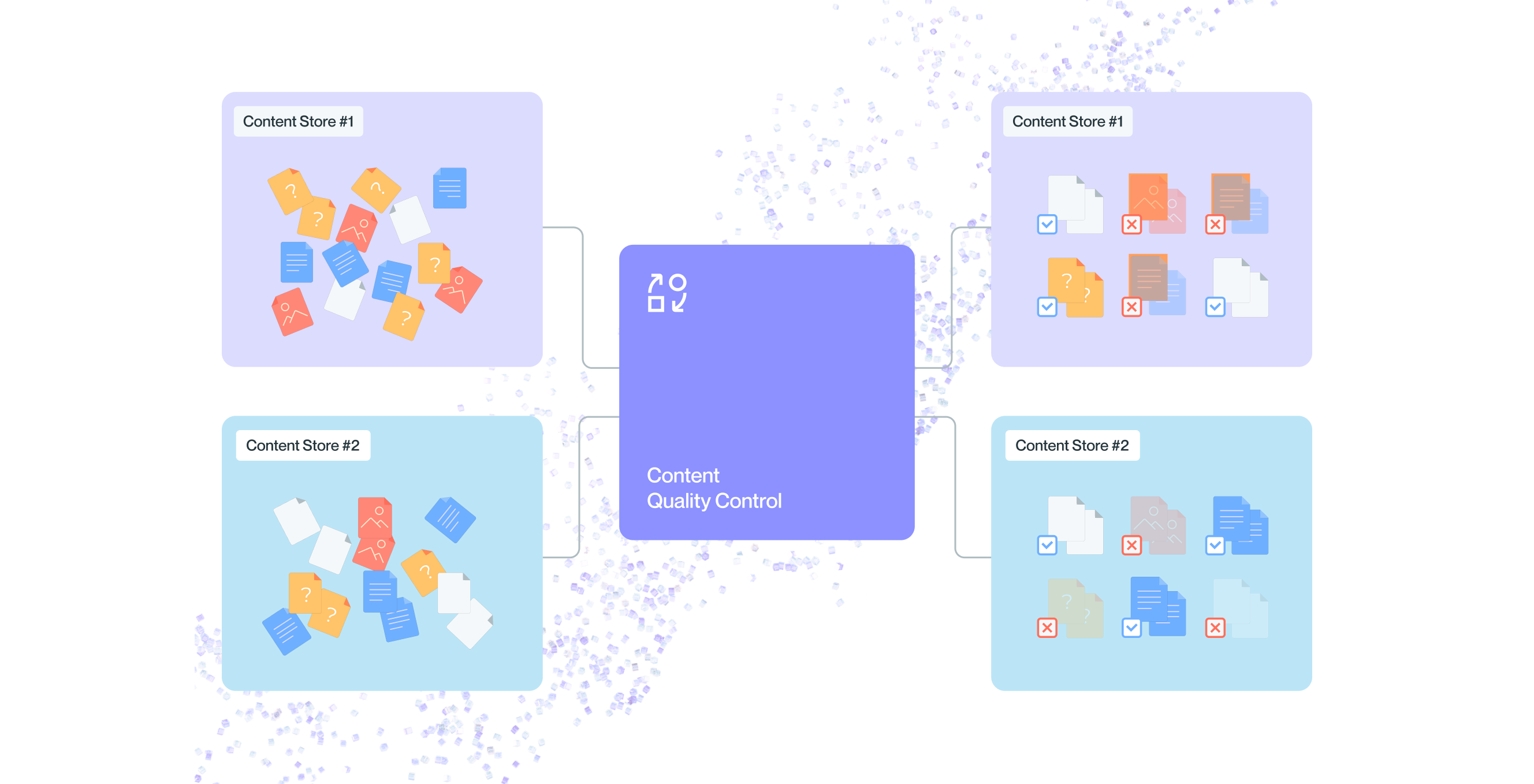
Unstructured data management is this needed quality layer. It is the practice of enriching, analyzing, cleaning, filtering and continuously monitoring the health of unstructured data. Developing an Unstructured Data Management strategy can help organizations improve and refine the content that gets consumed by both people and AI ensuring accurate, trusted answers everywhere they’re needed.
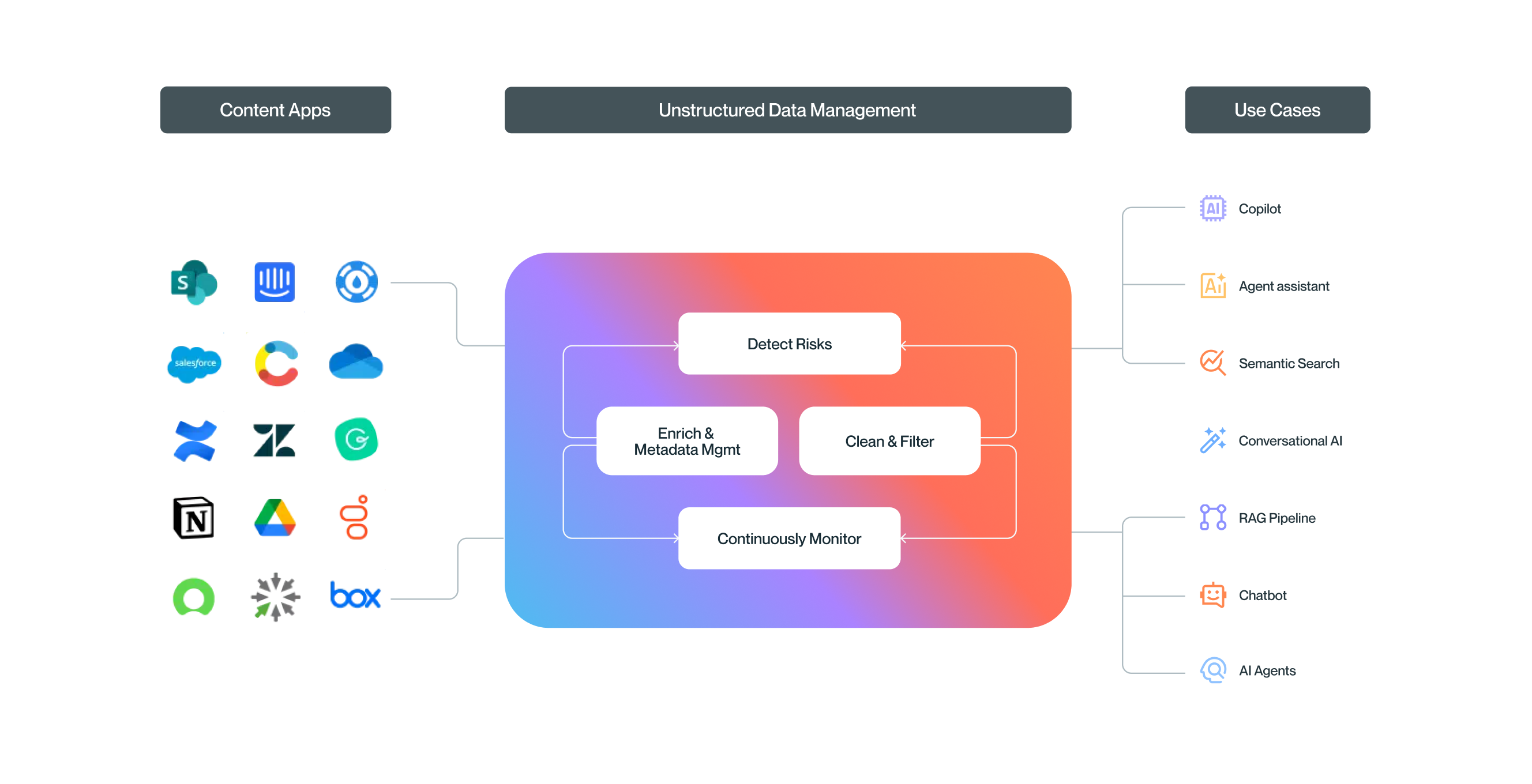
Some of the core tenets
of unstructured data management are:
Sync content from any source, transform it into an LLM-native format, and centralize it for processing – so that different content types, formats, and sources can be processed as a collection.
Enrich and standardize the metadata so that collections of unstructured data can be compared and assessed.
Analyze content collections to identify key risks, such as: conflicts, duplicates, out-of-date sections, and unstructured data gaps.
Control what data gets passed on to AI or Search, by filtering out risks or fixing incorrect, conflicting, or duplicate data.
Monitor the ongoing accuracy of unstructured data and get alerts on key risks, such as: compliance, toxicity, hallucinations, conflicts, and missing unstructured data.
Why Shelf
Shelf offers the world’s most advanced unstructured data management platform. Shelf was founded on the principle that knowledge is the life blood of an organization, the source of its competitiveness, innovation, know-how, and core competencies. The founding team has over 40 years of experience in knowledge management and unstructured data. Shelf’s mission is to empower humanity with better answers everywhere.
About usintro to Shelf.
.png)