As companies work to ensure the accuracy, compliance, and ethical alignment of their AI systems, they are increasingly recognizing the importance of AI audits in their governance toolkits.
What Is an AI Audit?
An AI audit is a comprehensive examination of an AI system that scrutinizes its architecture, implementation, and performance against predefined benchmarks. These audits are crucial for certifying that AI systems—aside from fulfilling their designated roles—adhere to ethical standards and regulatory requirements. The scope of an AI audit can extend from technical system accuracy to broader implications of usage, impacting stakeholders across the spectrum. At the core of a comprehensive AI audit are these four key pillars:
1. Analyzing AI Models, Algorithms, and Data Sources
Engines are at the core of AI systems – models and algorithms that process extensive data to make informed decisions or provide insight. A thorough AI audit investigates these components, checks the data inputs for authenticity and unbiased nature, and ensures the models operate on accurate and equitable data sets. This includes inspecting the integrity of data sources and the methodologies used for training AI models.
2. Technical Precision for Result Accuracy
Focusing on the precision and reliability of an AI system’s outputs, the technical audit confirms that the system’s performance aligns with the intended algorithmic design and data inputs. This part of the audit ensures that the AI delivers consistent and replicable results under similar operational conditions, maintaining a high standard of accuracy and reliability in its outputs.
3. Ethical Dimensions: Fairness, Legality, and Privacy
Evaluating an AI system’s ethical dimensions entails confirming that the system’s operations respect individual rights, comply with legal standards, and uphold privacy guidelines. This involves a thorough review of the decision-making frameworks used by the AI system in order to guarantee that there is no inherent bias in how decisions are made and that all operations are transparent and accountable. If applicable, compliance with international and local data protection regulations is also verified.
4. Continuous Operational Insights and Anomaly Detection
It’s essential to assess whether an AI system consistently meets its objectives and generates accurate outcomes. By analyzing on an ongoing basis the outputs it generates, and searching for anomalies, watch for signs that the AI systems’ performance is deviating from its expected course. This can enable identification of potential issues before they escalate.
5. Drift Detection
Drift detection involves monitoring the ongoing performance of AI systems to identify deviations that could impact accuracy. Model drift occurs when an AI model’s predictive accuracy changes over time due to shifts in behavior or the environment. Data drift happens when the statistical properties of the input data shift, affecting model performance. Timely identification of these drifts is crucial. Corrective strategies, such as retraining models or adjusting algorithms, ensure the AI system maintains reliability and effectiveness. Effective drift detection allows organizations to adapt swiftly to changing conditions, safeguarding the integrity of their AI solutions.
Pre-Audit Preparation
Several key activities that should precede a systematic audit to establish a strong foundation for a thorough and effective evaluation.
Prioritizing AI Systems for Audit
Prioritization helps focus resources on areas where the audit can provide the most valuable insights and assurances.
System Criticality
Systems that play a central role in high-stakes areas—such as financial forecasting, health diagnostics, or critical infrastructure operations—should be prioritized. The rationale is clear: a malfunction or bias in these systems could lead to significant operational disruptions or strategic missteps, making them prime candidates for scrutiny.
Algorithm Complexity
Systems built on complex algorithms, such as those involving deep learning or extensive neural networks, require more attention. These systems are not only harder to interpret (i.e., the ‘black box’ issue), but are also more susceptible to hidden biases and subtle computational errors.
Impact on Stakeholder Trust and Compliance
AI systems that manage or process sensitive personal data, influence customer interactions, or require adherence to specific regulatory frameworks (e.g.GDPR for data privacy or industry-specific regulations) are clear candidates for audits.
Determining Audit Objectives and Scope
Those tasked with pre-audit AI preparation must pinpoint exact goals and expansively delineate the audit’s terrain, guided by the dynamics and potential vulnerabilities inherent in AI systems.
Detailed Audit Objectives
Objectives for an AI audit are set with precision to thoroughly assess various critical aspects of AI operations:
- Performance Validation: This includes detailed evaluations of the AI’s operational accuracy and efficiency against designed benchmarks under varied conditions, assessing its resilience and adaptability.
- Safety Protocols Evaluation: The focus here is on examining the system’s mechanisms for handling errors and anomalies, assessing the robustness and reliability of these protocols to ensure they activate appropriately under abnormal conditions.
- Regulatory and Ethical Adherence: In order to audit AI, and ensure strict adherence to all applicable regulations and ethical guidelines, one requires a detailed review of compliance across data handling, user privacy, and transparency of AI decisions, especially under diverse international standards and regional regulations.
Expanding the Audit Scope
The scope of an AI audit should be a reflection of an AI audit checklist: expansive yet focused, targeting specific areas of the AI lifecycle while considering the system’s broader operational context:
- Comprehensive Lifecycle Review: Each phase of the AI lifecycle is examined systematically. This includes analyzing the integrity and security of data collection processes, the accuracy and fairness of model training, and the effectiveness of deployment and maintenance practices.
- Integration and Interaction Analysis: The integration of the AI system with existing IT frameworks and its interaction dynamics with users are evaluated. This involves assessing compatibility issues, data flow integrity, and the user experience to ensure seamless functionality and security.
- Risk-Focused Examination: The most critical components and processes within the AI system are identified for review. Areas with significant operational risk or where failure could lead to major repercussions are prioritized, applying a risk management framework to guide the auditing efforts effectively
Engaging with AI Governance Frameworks
The value of established AI governance frameworks and AI audit checklists in conducting an effective AI audit cannot be overstated. These frameworks are particularly beneficial when auditors need a systematic, standardized approach to examine complex AI systems. More than a set of guidelines, they can offer a comprehensive methodology that aligns AI operations with broader business and regulatory expectations.
Examples of such auditing frameworks are COBIT® 2019 and ISO/IEC standards.
COBIT® 2019 is often chosen for AI audits because it helps auditors assess whether AI initiatives are effectively governed, risk-managed, and controlled within the organization, providing a clear structure for ensuring that AI systems contribute positively to the business while managing potential risks prudently.
ISO/IEC are international standards that are known for setting benchmarks in data protection, security measures, and system integrity. By employing these standards, auditors can rigorously evaluate the AI’s compliance with global data security and quality norms, ensuring that the systems are not only effective but also secure and reliable across different jurisdictions.
The AI Audit Process
When the i’s are dotted and the t’s are crossed in the pre-audit preparation phase, the AI audit can smoothly transition into a methodical and disciplined examination process.
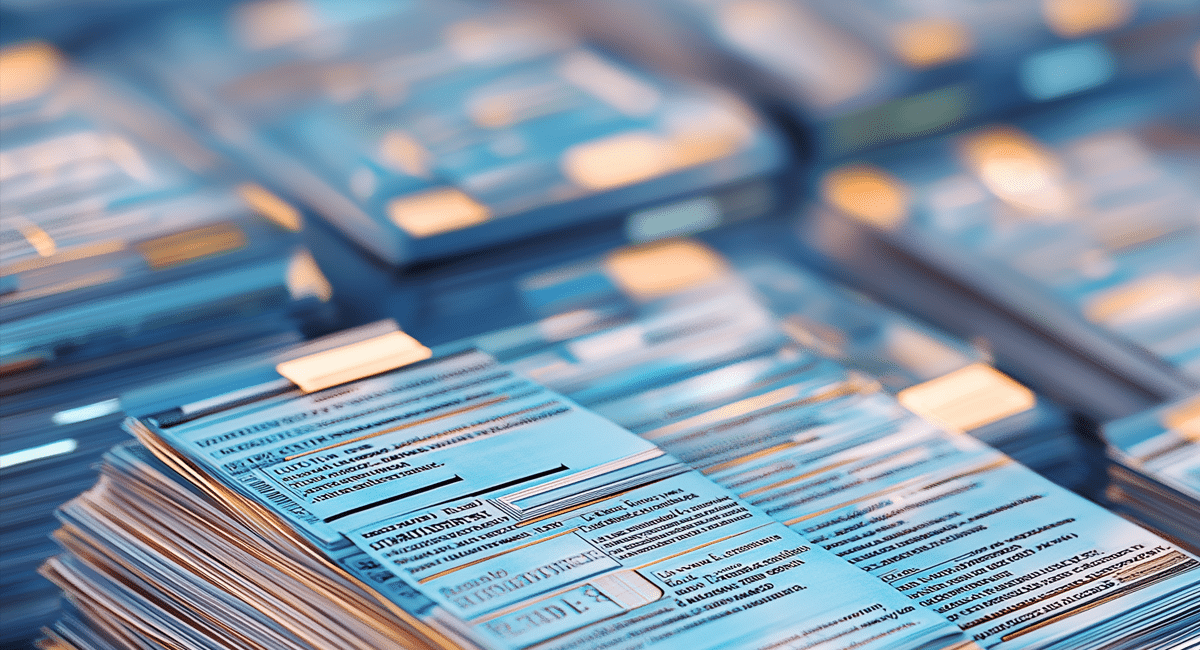
Planning Phase
The AI audit process begins with a meticulous approach to defining the audit criteria and methodologies, ensuring that each element of the AI systems under review will be thoroughly scrutinized against specific standards and expectations. Here, the auditor establishes the parameters that will guide the entire audit process, adapting generic frameworks to the unique aspects of the AI technologies implemented by the organization.
Defining Detailed Audit Criteria and Methodologies
The first step is to define audit criteria that precisely match the specific functions and potential risks of the AI systems. Instead of using a generic approach, the audit criteria should be customized to reflect the unique characteristics of each AI application. These criteria are adapted from flexible frameworks but are tailored as needed to fit the operational realities of the specific AI system.
The audit methodologies selected must be capable of uncovering the core of AI systems’ operations. This could involve a combination of quantitative analyses (i.e., performance metrics and data integrity checks), alongside qualitative methods such as stakeholder interviews and operational reviews.
Each method is carefully chosen to provide detailed insights that will ultimately keep the balanced and comprehensive audit approach.
Schedules and Resources
With the ‘what’ and ‘how’ clearly outlined, the focus shifts to ‘when’ and ‘who’. Each phase on the way to successfully auditing AI is carefully plotted on the timeline, ensuring ample space for thorough analysis, unexpected discoveries, and thoughtful conclusion drawing.
Resource allocation focuses on assembling an audit team with diverse expertise—ranging from AI technology specialists and data scientists to ethical compliance and cybersecurity experts. This team will conduct the audit but also interpret the findings, and question and challenge them.
Execution Phase
The execution phase is where concept meets concrete. Auditors search through the operational layers of AI systems, collecting data, evaluating practices, and testing the systems’ outputs to validate their integrity and effectiveness.
Data Collection
The first task in this phase is the systematic collection of data regarding the AI systems’ processes, algorithms, and the datasets used for training. This involves not just the extraction of technical data, but also understanding the context in which the data operates. Auditors gather comprehensive details about the architecture of the AI systems, the nature of the data they process, and the mechanisms by which they evolve and learn.
Evaluation
With data in hand, the focus shifts to evaluation of the data against a spectrum of criteria:
- Internal Controls and Compliance Review: Auditors scrutinize the systems for adherence to financial, privacy, and operational standards that govern their deployment. This includes checking for compliance with global regulations such as GDPR for data protection or specific industry mandates that dictate how AI must be managed.
- Fairness Analysis: This step involves dissecting the AI’s decision-making process to detect and address any biases that might skew outputs. Techniques like analysis of variance within decision metrics help auditors identify any discriminatory patterns that could affect fairness.
- Transparency and Accountability Measures: The clarity with which AI systems operate need to be placed underneath a microscope. Auditors assess whether AI systems are equipped to explain their decisions in understandable terms. This includes reviewing the documentation and logic paths that detail how and why decisions are made.
- Ethical Integrity Checks: Auditors evaluate the ethical dimensions of AI deployment. This involves ensuring that AI operations align with ethical principles such as respect for user privacy, integrity, and fairness. AI systems must be designed to respect user rights and feature mechanisms that are meant to correct any ethical missteps.
- Review of Decision Frameworks: Lastly, the structure and rationale behind AI decision-making are reviewed. This detailed analysis looks at how decisions are formulated within the AI systems, checking for consistency, the potential for human oversight, and the mechanisms for feedback and improvement.
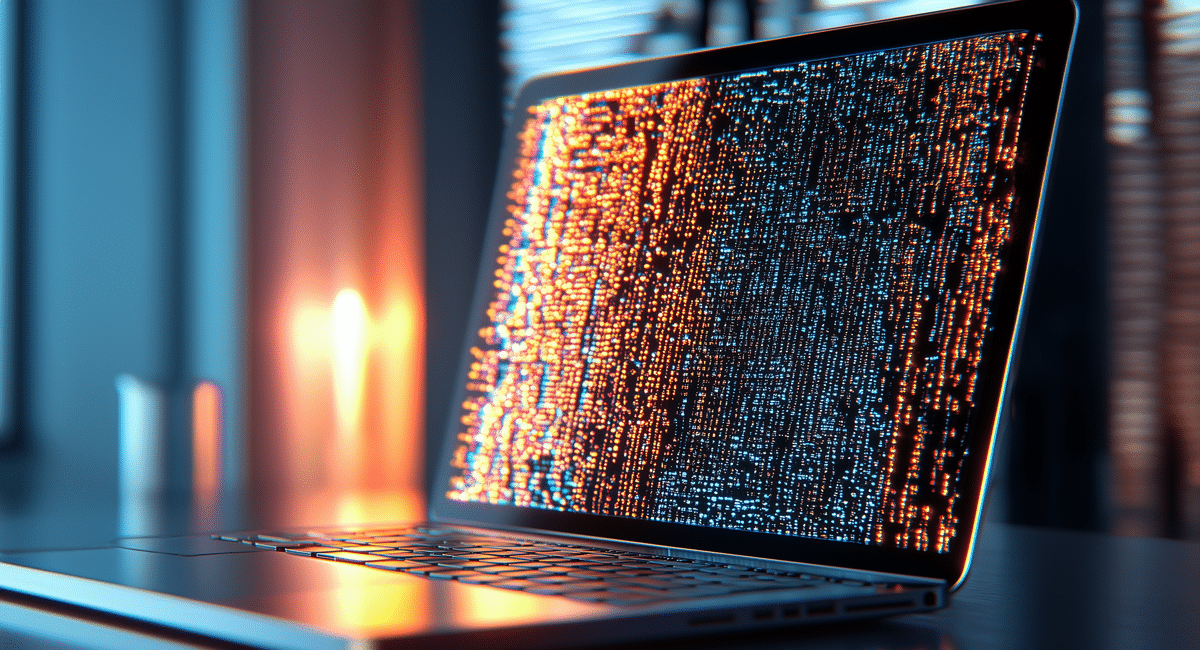
Testing
The task of testing is to confirm that the outputs of AI systems are accurate and dependable. This involves a suite of specific tests tailored to subject the systems to a variety of conditions, ensuring they consistently deliver quality results:
- Accuracy Checks: The first step usually includes detailed accuracy checks where AI outputs are compared against established benchmarks or expected results. One way to run such a test is to check the AI against a set of control data where the outcomes are already known, allowing auditors to measure how well the AI’s predictions or decisions align with reality.
- Integrity Trials: Here, auditors implement trials that test the AI’s output robustness across varying operational conditions and over time. For instance, introducing perturbations or noise into the input data to see if the AI maintains its performance can be particularly revealing.
- Consistency Evaluations: This aspect examines the AI system’s ability to deliver the same outputs under similar circumstances consistently, a key factor in a system that has to be reliable at all times.
- Scenario Testing: To gauge how the AI handles unexpected or rare data scenarios, auditors use tailored tests designed to challenge the AI with atypical or extreme data sets. This helps identify potential vulnerabilities or areas where the AI might not perform optimally.
- Traceability Verification: A vital part of testing is ensuring that AI decisions can be traced and justified. This involves verifying that each decision made by the AI can be audited and traced back to specific data points and decision-making processes.
Reporting Phase
The report begins by summarizing the results obtained from the various tests and assessments performed during the execution phase. The result is a narrative that lists findings and probes into the reasons behind anomalies or deviations from expected behaviors. It explores the implications of these findings in a way that contextualizes their impact on both the AI system and its broader operational environment.
Combine Technical Scrutiny with Established Frameworks
In order to audit AI as thoroughly as possible, one needs a combination of rigorous technical scrutiny with an acute awareness of ethical standards and compliance frameworks. It is a comprehensive endeavor that extends beyond mere operational assessments to include evaluations of ethical, legal, and privacy considerations. Performing a thorough AI audit is a long path, from pre-audit preparations through to the execution and reporting phases, on the way to ensure AI systems function as they are intended to.
AI audits provide the needed assurance that these systems operate without bias, adhere to ethical principles, and comply with regulatory standards. They are the promoters of transparency, building trust among users and stakeholders by demonstrating that the AI systems are accountable and their operations are justifiable.